Exploring the Synergy of Big Data, AI, and ML
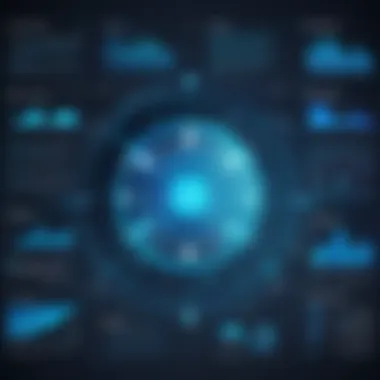
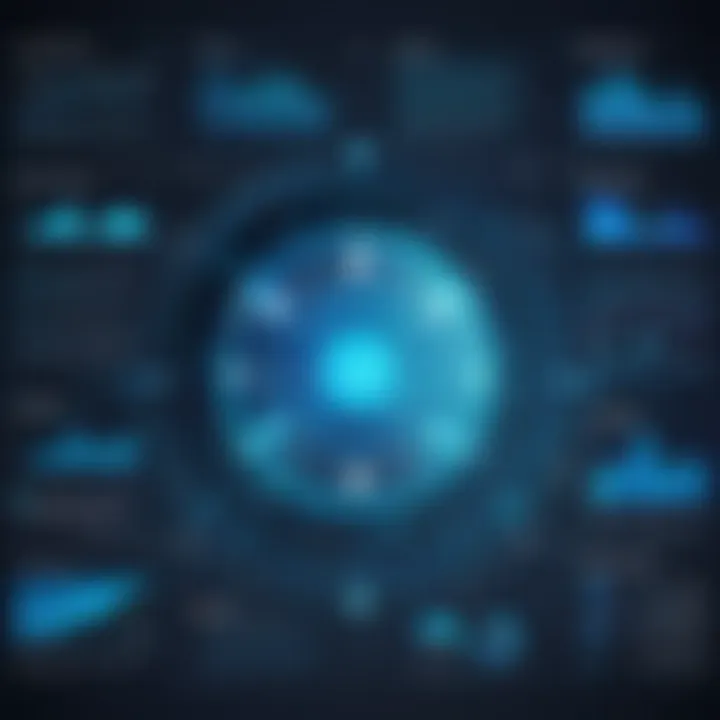
Intro
In a world inundated with data, understanding the synergy between big data, artificial intelligence (AI), and machine learning is not just a luxuryâit's a necessity. This trifecta is reshaping industries, fostering innovation, and driving efficiency in ways we couldn't have imagined just a decade ago. Think about it: every click, swipe, and scroll generates a wealth of information. This trove of data, when harnessed properly, can lead to groundbreaking advancements in various domains, from healthcare to finance.
As we embark on this exploration, we will unpack the intricacies of each component, how they interlink, and their implications for the future. The relationship between these technologies is complex, filled with opportunities and challenges alike.
The Role of Big Data
Big data refers to the vast volumes of data generated at unprecedented speeds. Itâs not just about size; the variety of dataâfrom structured numbers to unstructured text and imagesâmakes it imperative for organizations to develop the right tools to process and analyze it. Companies like Netflix and Amazon excel at utilizing big data to tailor their offerings, ensuring a personalized experience for each user.
However, storing and managing such an enormous amount of data brings certain challenges. Data privacy concerns have risen, especially as regulations like GDPR set stringent guidelines on data usage. So, while the potential is vast, thereâs always a need to tread carefully, ensuring ethical standards are upheld.
AI and Its Connection to Big Data
Artificial intelligence can be seen as the brain that interprets big data. Algorithms powered by AI sift through mountains of information, identifying patterns and making predictions that humans might miss. For instance, in healthcare, AI systems analyze patient histories alongside new research data to suggest treatment plans or identify early signs of diseases.
The relationship is reciprocal; as big data grows, AI technology must evolve to handle it effectively. This cycle enhances predictive analytics, making businesses smarter and more efficient in their operations.
Machine Learning: The Learning Aspect
Machine learning is a subset of AI more focused on enabling systems to learn from data autonomously. Imagine a smart system that continuously refines its performance as it processes more information. For example, consider the recommendation algorithms used by various streaming platforms. They watch your behavior and preferences, gradually honing in on what you might like next.
In sectors like finance, machine learning has revolutionized processes, from fraud detection to credit scoring. By analyzing consumer behaviors and historical data trends, systems can make real-time risk assessments, helping institutions stay a step ahead of potential threats.
Ethical Challenges
With great power comes great responsibility. As we delve deeper into this data-driven era, ethical issues loom large. Transparency in algorithms is criticalâstakeholders must understand how decisions are made. Moreover, potential biases embedded in algorithms can have profound impacts, inadvertently perpetuating stereotypes or excluding marginalized groups.
Enter data ethics, a budding field aimed at addressing these concerns. It's becoming increasingly clear that reliance on AI without careful consideration can lead to unintended consequences. Organizations are urged to prioritize ethical frameworks to guide their data practices moving forward.
Epilogue
The interplay of big data, AI, and machine learning is a complex dance. Organizations that can adeptly navigate this landscape will not only reap the benefits of innovation but will also set the stage for a more efficient, equitable digital future. As we further untangle this multifaceted thread, it's crucial to remain vigilant about the challenges that accompany such rapid advancements.
Implying a forward-looking approach that integrates innovation with intentionality is vital for making the most of these technologies. The future is indeed bright, but it watches closely from the shadows, reminding us of our responsibility to manage it wisely.
Understanding Big Data
Grasping the essence of big data is essential in todayâs rapidly evolving technological landscape. It's not merely about having access to a massive amount of information; itâs about how that information can be harnessed to create value. Understanding big data lays the groundwork for comprehending the relationship it shares with artificial intelligence (AI) and machine learning (ML). The concepts explore how organizations can leverage data insights for decision-making, predictive analytics, and personalized experiences.
Defining Big Data
Big data refers to the vast volumes of structured and unstructured data generated every second from various sources. This phenomenon has transformed how we analyze and interpret information. It's not just the size that matters; itâs also the complexity and the speed at which this data is generated. For instance, companies like Facebook gather terabytes of data daily, which can reveal trends, consumer preferences, and behaviors. Therefore, defining big data involves recognizing its multifaceted nature and the impact it has across industries.
Characteristics of Big Data
The characteristics that define big data can generally be summarized by the five Vs: Volume, Velocity, Variety, Veracity, and Value. Each of these plays a significant role in shaping how businesses approach data and analytics.
- Volume:
Volume signifies the sheer amount of data generated. It is one of the most apparent aspects associated with big data. The massive scales of data generated through social media, transactional records, and online activities demand robust storage solutions and analytical tools. Keeping track of millions of transactions or interactions is a complex but necessary task for businesses today. The larger the volume, the more potential insights available; however, it also brings challenges in data management and analytics. - Velocity:
Velocity signifies the speed at which data flows. With real-time data streaming from social media feeds and IoT devices, analysis must happen at lightning-fast speeds. Whether itâs a stock trading algorithm or a real-time recommendation system, the capacity to process data swiftly allows organizations to make informed decisions. Although this rapidity offers the chance to act promptly, it can also lead to issues if data is not correctly filtered or analyzed, resulting in poor decision-making. - Variety:
Variety highlights the different formats and sources of data. Data can come from various avenuesâtext, images, videos, and more. In a world abundant with numerous forms of information, being able to integrate and analyze various types is crucial. The presence of such diversity allows for a comprehensive understanding of customer preferences, although managing different types requires advanced tools. - Veracity:
Veracity refers to the quality and accuracy of the data. Not all data generated is of good quality; much of it can be inconsistent, ambiguous, or biased. Ensuring high veracity is vital for effective analysis. Low-quality data can mislead insights, resulting in erroneous predictions and decisions. Organizations must therefore develop strong mechanisms for data validation to ensure reliability. - Value:
Lastly, value represents the significance and actionable insights derived from big data. Simply gathering immense volumes of data doesnât equate to value. Organizations need to focus on extracting actionable insights that culminate in improved decision-making. Data without context is just noise, and distinguishing whatâs valuable is key.
Sources of Big Data
Organizations gather big data from numerous sources, each contributing uniquely to the overall data landscape. Understanding these sources is important for accurately capturing and analyzing the data.
- Social Media:
Social media platforms like Facebook, Twitter, and Instagram are treasure troves of user-generated content. They disclose insights on consumer behavior, preferences, and trends. Analyzing patterns from social media interactions can greatly assist businesses in tailoring marketing strategies. However, the vastness and unpredictable nature of social media data can complicate analysis and may require advanced tools for effective interpretation. - IoT Devices:
The rise of IoT devices contributes significantly to data generation. From smart home gadgets to wearable fitness trackers, every device continuously produces data. This enables businesses to monitor behavior in real time, creating opportunities for tailored services and predictive maintenance. While valuable, it also presents challenges in terms of security and data management. - Transactional Databases:
Every transaction executed in retail stores, e-commerce platforms, and financial institutions generates data. Transactional databases are excellent sources for understanding purchasing patterns and financial behaviors. Nevertheless, analyzing these databases requires robust SQL skills and may introduce complexities due to inconsistent data entries. - Sensor Data:
Sensors collect data from various environments, delivering real-time information. Whether in agriculture, manufacturing, or healthcare, sensor data provides insights that can optimize operations. For instance, agriculture sensors can monitor soil conditions for better crop management decisions. The challenge, however, lies in gathering, integrating, and interpreting the data effectively.
The Role of Artificial Intelligence
Artificial Intelligence (AI) plays a crucial role in not just the development of technology, but in transforming how we interact with the world. For many, AI represents the pinnacle of innovation, a sort of magic wand that can draw insights, predict outcomes, and automate laborious tasks in ways previously only imagined. In the context of this article, itâs vital to unpack how AI interlinks with big data and machine learning, driving efficiency and unleashing new avenues of knowledge and operation across various sectors.
AI: An Overview
AI can be thought of as the overarching branch of computer science that aims at creating systems capable of performing tasks that require human intelligence. This encompasses anything from simple rule-based processes to more sophisticated neural networks that learn from data. The beauty of AI lies in its adaptability; it evolves based on the information fed into it, allowing for near-infinite applications.
AI Techniques and Algorithms
Natural Language Processing
Natural Language Processing, commonly abbreviated as NLP, stands as a bridge between human language and computer understanding. It allows machines to process and understand large amounts of natural language data, which is crucial in an environment rich in informationâthe digital landscape. One key characteristic of NLP is its ability to glean meaning from context, something that enhances user interactions with technology. A notable contributor means developers can automate customer service tasks, doing away with the long waits often associated with traditional methods. Though highly effective, it can also face challenges related to language nuances and ambiguities, requiring continuous improvement in its algorithms.
Computer Vision
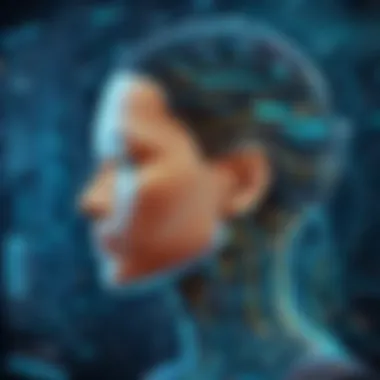
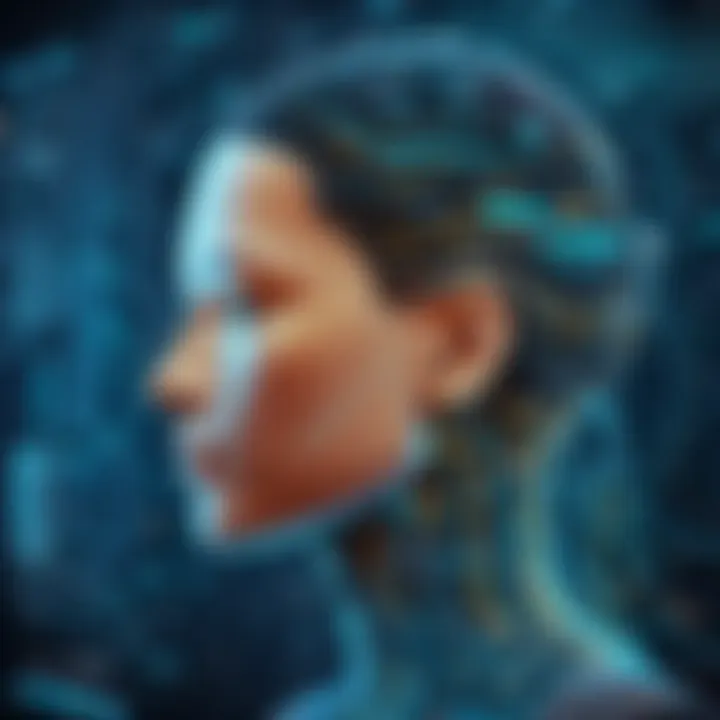
Computer Vision enables machines to interpret and make decisions based on visual inputs. The focus here is on replicating some components of human sight in a digital format. A critical aspect is its application in fields like security and automotiveâwith real-time processing of images, enhancing safety measures or powering self-driving cars. This technology is a game changer, though it inherently struggles with variability in lighting and environments, thus calling for robust datasets to train models effectively.
Predictive Analytics
Predictive Analytics uses statistical algorithms and machine learning techniques to identify the likelihood of future outcomes based on historical data. It provides businesses with foresight, a powerful tool for decision-making. A significant benefit of this approach is in trend analysis, allowing companies to pivot strategies based on projected consumer behavior. However, this technique relies heavily on the quality of data; poor data can lead to misleading predictions, and thus, emphasizes the need for strong data governance.
Applications of AI in Various Sectors
Healthcare
In healthcare, AIâs capabilities are unmatched. By analyzing complex medical data, AI can assist in diagnostics, predict patient outcomes, and even personalize treatment plans. This characteristic of precision enhances the reliability of healthcare decisions. A unique aspect is its application in genomicsârecognizing patterns in genetic data to identify predispositions for specific conditions. However, the challenge lies in ensuring that ethical standards are upheld to protect patient data privacy.
Finance
The finance sector increasingly leans on AI for tasks like fraud detection and risk management. The ability to quickly analyze transactions and identify suspicious activities is a significant boon. Another key feature is algorithmic trading, where decisions are made at lightning speed based on real-time data. However, over-reliance on such systems poses risks, as unforeseen market changes could lead to substantial losses if the algorithms are not robust enough.
Retail
AIâs application in the retail sector is all around enhancing customer experience. From personalized recommendations to inventory management, AI tailors services to consumer preferences. A compelling aspect is its role in analyzing customer feedback to improve services. On the downside, the challenge here is balancing automation with a personal touch, where too much reliance on AI can lead to a loss in customer satisfaction if the human element is overlooked.
Manufacturing
Manufacturing benefits from AI through automation and optimization. Smart factories utilize AI to streamline operations, reduce waste, and improve efficiency. One noted feature includes predictive maintenance, where machines self-report issues before breakdowns occur, saving time and costs. The downside, though, isnât insignificant; thereâs a growing fear about job displacement as automation rises, needing consideration of potential workforce impacts.
"AI is not just a tool; it's becoming part of the decision-making process across all sectors, reshaping our relationship with technology."
Through the various dimensions highlighted, it's clear that AI is not merely an add-on in today's digital landscape but a central figure driving innovation and operational effectiveness.
Machine Learning Explained
Machine learning is often touted as a cornerstone of artificial intelligence and an integral part of the broader big data landscape. Understanding it helps demystify how machines decipher complex patterns from vast amounts of data. With every advancement in data analytics, the relevance of machine learning grows, making it vital for professionals in tech. Here, we will delve into the essentials of machine learning, touching upon its types and the workflow that guides it.
Machine Learning Basics
At its core, machine learning is about teaching computers to learn from data. Instead of relying on hard-coded instructions, machines analyze information and adapt their performances based on new inputs. This adaptability is a key reason machine learning shines in various applications, from recommendation systems to fraud detection. By harnessing historical and real-time data, these systems improve over time.
A crucial point is that unlike traditional programming, where a programmer specifies exact steps to solve a problem, machine learning requires collecting enough data for the machine to make educated decisions on its own. This shift to data-driven algorithms represents a paradigm change in how we approach problem-solving.
Types of Machine Learning
Across the landscape of machine learning, we generally categorize it into three main techniques: supervised learning, unsupervised learning, and reinforcement learning.
Supervised Learning
Supervised learning is like having a mentor guide you while you learn a new skill. In this scenario, a model is trained using labeled datasets, where the input data is paired with the correct outputs. This approach shines in applications like image recognition or email classification, where knowing the correct outcome improves the model's predictions.
The biggest advantage of supervised learning is its ability for precise training, making it a popular choice for developers. However, it comes with a catch. Building and curating accurate labeled datasets can be time-consuming and sometimes costly. The reliance on human-provided labels introduces a potential biasâif the training data isn't representative, the model's predictions can suffer.
Unsupervised Learning
On the flip side, you have unsupervised learning. This technique is the quiet observer, analyzing the data without any guidance on what to look for. Here, the model works with unlabelled datasets to uncover hidden structures and patterns. Think of it like exploring a dense fog; the model starts identifying clusters and relationships without any prior context.
A standout benefit of unsupervised learning is its ability to generalize, making it great for tasks like market segmentation or anomaly detection. However, the lack of clear objectives can also be limiting; sometimes the insights gleaned may lack clarity or relevance to business needs.
Reinforcement Learning
Reinforcement learning, the last of the trio, mimics a trial-and-error approachâlike training a pet. In this setup, a model learns through a system of rewards and punishments based on its actions in a given environment. It's particularly notable in applications like robotics, game playing, and self-driving cars, where reactive decision-making is key.
The crux of reinforcement learning lies in its experiential learning; it continually refines its strategies based on successes and failures. However, this can lead to slower training times and significant computational resource requirements, which might deter its adoption in certain projects.
The Machine Learning Workflow
To fully appreciate machine learning's capabilities, one must understand the workflow designed to harness its power effectively. This entails several stages: data collection, data preprocessing, and model training.
Data Collection
That's where the journey begins. Data collection is the very foundation of any machine learning project. The quality and volume of data gathered directly impact the model's performance. Sources vary widely, from IoT devices and social media platforms to enterprise data warehouses.
The key characteristic of effective data collection is identifying relevant datasets that provide a comprehensive view of the problem at hand. If done right, this stage lays the groundwork for the entire project. However, the challenge lies in dealing with potential data quality issues and ensuring sufficient diversity to avoid bias.
Data Preprocessing
Once data has been gathered, it needs to be cleaned and transformed. This is where data preprocessing comes into play. It involves steps like removing duplicates and dealing with missing values, which ensures a clean dataset for training.
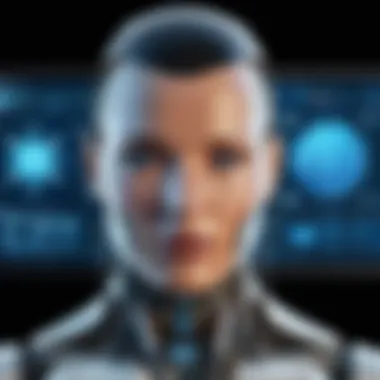
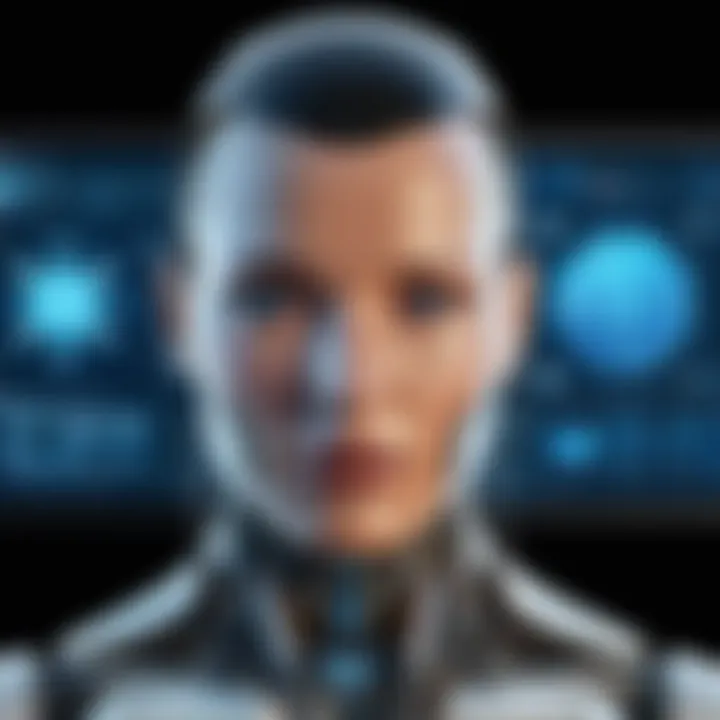
The importance of this step cannot be overstated; problems in data preprocessing can lead computers down the wrong path, resulting in faulty models that yield incorrect predictions. This stage is where many beginners fall flatâoversights here can throw a wrench into the works.
Model Training
After preprocessing, itâs time to train the model. During model training, algorithms learn to generalize from the input data. The goal is to minimize error in predictions, and this often involves adjusting model parameters through various techniques, including cross-validation or grid search.
Training can be resource-intensive, demanding robust hardware and time, yet this is the stage where the model evolves. Itâs not merely a mechanical process; the intuition behind tweaking parameters draws on data science prowess. If successful, the outcome will manifest as a model capable of making informed predictions.
In summary, machine learning isnât just some buzzword floating in tech culture; it represents a transformative elixir in the world of data. Its multifaceted approaches provide robust strategies for different challenges, aiming to refine decision-making processes and propel innovation across industries. Whether you're an experienced programmer or new to the field, understanding machine learning equips you with the necessary tools to navigate the complexities of the digital era.
Big Data and AI: A Synergistic Relationship
In todayâs digital age, the relationship between big data and artificial intelligence (AI) stands as a cornerstone for innovation and development. Big data serves as the bedrock upon which AI models are built, enabling them to learn and refine their processes. The interdependence between these two entities can be likened to a well-oiled machine; each part works in harmony, amplifying the capabilities of the other. Understanding how big data fuels AI is pivotal in grasping how these technologies are transforming industries across the board.
How Big Data Fuels AI
At the heart of AIâs effectiveness is the sheer volume of data available for processing and analysis. Big data â characterized by its vastness and complexity â provides the raw material necessary for machine learning algorithms to learn, adapt, and improve. Without this resource, AI models would be akin to a ship lost at sea; directionless and unpredictable.
AI algorithms require significant amounts of training data to deliver meaningful outcomes. For instance, in natural language processing, large datasets help models like OpenAI's GPT refine their understanding of human language, ultimately enhancing their ability to generate coherent text. Similarly, in image recognition, AI systems sift through millions of labeled images, identifying patterns and features that enable them to differentiate between objects with remarkable accuracy.
Moreover, the dynamic nature of big data enables AI systems to adapt and maintain relevance in a world that is constantly evolving. Consider, for example, how Netflix utilizes big data to analyze viewer behaviors. By correlating viewing habits with user data, Netflix enhances its recommendation algorithms, significantly improving user experience and engagement. This kind of practical application showcases how big data directly impacts AI capabilities, making them more responsive and tailored to individual needs.
AI Enhancements through Big Data Analytics
The role of big data analytics in enhancing AI cannot be overstated. It allows organizations to extract insights from massive datasets, creating a feedback loop that further enriches AI algorithms. Through these analyses, companies can unearth trends and correlations that inform decision-making processes.
"Data is the new oil. Itâs valuable, but if unrefined it cannot really be used."
â Clive Humby
Several sectors, including finance, healthcare, and retail, leverage big data analytics to drive strategic initiatives. In finance, for instance, data-driven models predict market trends, allowing traders to make informed decisions and manage risks effectively. The healthcare sector benefits tremendously by screening large datasets for patterns associated with diseases, paving the way for more accurate diagnostics and individualized treatment plans.
Additionally, big data analytics creates opportunities for continuous learning within AI systems. By constantly feeding new data into existing models, companies can refine their algorithms, enhancing accuracy and performance over time. This sort of evolution is essential in an environment where user preferences are in constant flux.
In summary, the relationship between big data and AI is synergistic in nature, with each relying on the other to achieve operational excellence. Big data supplies the necessary resources for AI to learn and adapt, while AI employs these insights to enhance its analytics capabilities. This interplay not only drives technological advancements but also ensures a more responsive and personalized experience across various applications.
Machine Learning: The Driving Force Behind AI
In the realm of artificial intelligence, machine learning stands as a crucial pillar that transforms complex data into actionable insights. This section elucidates the roles and intricacies of machine learning, highlighting its foundational importance in AI's evolution. As industries pivot towards data-driven decision-making, understanding machine learning becomes imperative not only for its technical merits but also for the socio-economic implications it holds.
The Integration of Machine Learning with AI
Machine learning (ML) can be best understood as a subset of AI that focuses on enabling machines to learn from data and improve their performance over time without being explicitly programmed. This integration is where the real magic happens. By leveraging large datasets, ML algorithms develop models that can predict outcomes, classify data, and even generate new insights based on learned patterns.
- Enhanced Accuracy: ML algorithms enable AI systems to make predictions that are increasingly precise. For instance, in healthcare, machine learning models can analyze patient data to predict disease progression more accurately than traditional methods.
- Scalability and Adaptability: The marriage of ML and AI provides systems with the ability to continually learn and adapt in real-time. As new data streams in, these systems can re-calibrate, providing up-to-date insights without necessitating manual interventions.
- Automation of Decision-Making: Organizations benefit immensely from automating routine decisions. For example, companies utilize recommendation algorithms in e-commerce platforms to suggest products based on users' past purchases and preferences, thus enhancing customer experience.
The practical ramifications of this integration are profound. From self-driving cars navigating through traffic to intelligent virtual assistants managing household tasks, the versatility of machine learning empowers AI applications to operate more effectively across diverse scenarios.
Evolution of Machine Learning Algorithms
The trajectory of machine learning has been marked by significant breakthroughs that have propelled AI into new territories. Understanding this evolution illustrates the strides taken in algorithm development, optimization, and application.
- Early Algorithms: Beginning with foundational models like linear regression or decision trees, early machine learning focused on relatively simple datasets. However, as more data became accessible, these models required enhancements to manage complexity.
- Emergence of Deep Learning: The rise of deep learning, a specialized area of machine learning, marked a pivotal shift. Utilizing artificial neural networks, deep learning allows for the extraction of intricate features from vast datasets. This innovation has been particularly influential in fields such as image recognition and natural language processing.
- Continuous Improvement: Algorithms today are not static; they evolve. Techniques like ensemble learning, which combines multiple learning algorithms to enhance performance, have proven effective. For instance, a random forest model can outperform individual decision trees by aggregating their predictions, leading to greater accuracy.
"The evolution of machine learning isn't just about improved algorithms; it's about rethinking how we engage with data, making it teach machines what ultimately matters."
As the landscape of machine learning continues to grow, so too does its capacity to influence varied sectors like finance, health, education, and beyond. Each advancement opens doors to innovative applications, reshaping industries and driving efficiency in ways previously unseen.
Challenges in Big Data, AI, and Machine Learning
In this age where data is dubbed the new oil, addressing the challenges inherent in big data, artificial intelligence, and machine learning is crucial. These challenges possess the potential to hinder progress or, conversely, drive innovation when approached prudently. The interplay among these elements is not merely academic; it has real-world implications that affect businesses, consumers, and society at large.
Data Privacy Concerns
As data flows in vast torrents from various sources, it raises serious privacy concerns. With the advent of the Internet of Things and social media, sensitive personal information is often collected, analyzed, and utilized without sufficient transparency. Mismanagement can lead to significant breaches. It's no picnic when a consumer's data gets misused, as seen in notable cases like the Cambridge Analytica scandal.
The legal frameworks governing data privacy vary drastically across regions. For instance, the European Union's General Data Protection Regulation (GDPR) has stringent rules in place, contrasting with looser guidelines found elsewhere. Organizations grapple with not only collecting and processing data responsibly, but ensuring compliance as well.
"In the world of data, those who cannot protect their customers' information will find themselves out of business faster than you can say 'data breach.'"
Bias in AI Models
Bias in AI models can be a double-edged sword. Itâs essential to recognize that AI does not operate in a vacuum; it learns from existing data, which may reflect historical biases. Whether in hiring practices, loan approvals, or even law enforcement, biased algorithms can perpetuate inequities, adversely affecting marginalized communities.
For instance, facial recognition technology has been shown to misidentify individuals of certain ethnic backgrounds more frequently than others. This lack of a level playing field raises ethical questions, creating a need for diverse data sets along with algorithmic adjustments. Ensuring fairness, transparency, and accountability frameworks will be pivotal in addressing these biases.
Scalability of Machine Learning Models
The scalability of machine learning models directly correlates with the amount of data available. While large datasets provide the opportunity for growth, challenges inevitably arise when attempting to manage and process increasing volumes of data effectively. A model that functions well in a controlled environment may falter under the pressures of real-world complexities.

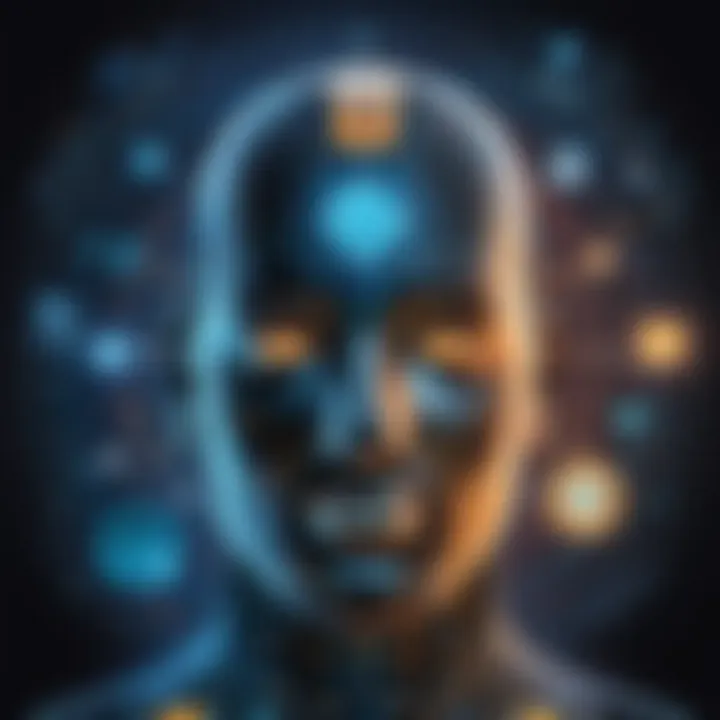
Consider a retail company that uses machine learning for inventory predictions. As they scale, the model must adapt to new product lines, seasonality, and historical customer behavior. This adaptability requires not only robust infrastructure but also continuous model training. Companies often find themselves in a bindâstriking a balance between high performance and cost-effectiveness when managing infrastructure resources.
Successful navigation of these challenges is vital for harnessing the full potential of big data, AI, and machine learning. By being proactive rather than reactive, organizations can foster a data-driven environment that emphasizes ethical use and practical scalability.
Ethical Considerations in AI and Data Utilization
The topic of ethical considerations in AI and data utilization sits at the very heart of our digital future. As big data and AI grow more entwined in the fabric of our society, understanding the implications of their utilization becomes essential. Ethical AI is not just a buzzword; it embodies the principles that should guide the development and implementation of these technologies. Through addressing these ethical concerns, stakeholders can harness the benefits of AI while minimizing potential harm.
Importance of Ethical AI
Ethical AI is vital for several compelling reasons.
- Trust and Acceptance: Users are more likely to embrace AI systems that operate transparently and fairly. When organizations prioritize ethical considerations, they build trust with users, enhancing overall acceptance of AI technologies.
- Preventing Harm: By analyzing potential risks in AI deployment, businesses can proactively avoid pitfalls that may arise from biased algorithms or data mishandling. The aim is to create systems that respect user privacy and data integrity.
- Legal Compliance: Different regions are implementing stringent regulations around data usage and privacy. Ethical AI paves the way for compliance with these regulations, thereby preventing legal repercussions for firms that might mismanage data.
Consider, for example, the case of a financial technology company deploying an AI-driven loan approval system. If that system inadvertently uses biased data, it may lead to discriminatory practices, denying loans to certain demographics unfairly. This not only harms individuals but also jeopardizes the companyâs reputation and legal standing.
Frameworks for Responsible AI
To promote responsible AI, various frameworks have been introduced. These frameworks provide a structured way to approach ethical considerations in AI design and implementation.
- Principles-based Frameworks: Many organizations adopt broad principles such as fairness, accountability, and transparency. These principles serve as guiding lights for the development process to maintain a focus on ethical implications.
- Impact Assessments: Conducting impact assessments before deploying AI models can help identify potential harms and unfair biases. Evaluating both the positive and negative impacts allows for informed decision-making.
- Collaboration with Stakeholders: Engaging diverse stakeholders, including ethicists, community members, and AI developers, can yield a more holistic approach to responsible AI practices. This collaboration ensures that multiple perspectives are considered, ultimately leading to more ethical outcomes.
"AI systems should not only operate effectively, but also align with the values and needs of society."
- Continuous Monitoring: The rapid evolution of AI demands ongoing scrutiny of deployed systems. Establishing mechanisms for monitoring AI performance post-deployment helps identify and rectify issues as they arise, ensuring continuous compliance with ethical standards.
In summation, ethical considerations in AI and data utilization are paramount to creating technology that positively impacts society. As we navigate the complexities of AI and big data, understanding these frameworks and the importance of ethical AI will lead us towards a more just and responsible digital landscape.
Future Trends in Big Data, AI, and Machine Learning
The landscape of technology is perpetually evolving, and the interplay among big data, artificial intelligence, and machine learning continuously transforms various sectors. Keeping an eye on emerging trends in these domains is crucial for anyone in the field. Understanding these trends not only helps professionals adapt but also empowers them to harness opportunities for innovation and efficiency. Let's delve into some key future trends that will shape the interaction between these technologies.
Advancements in AI Algorithms
As we look ahead, one of the most striking aspects is the rapid progression of AI algorithms. No longer is it just about training neural networks; weâre entering an era where algorithms are becoming smarter, more efficient, and adaptable. Researchers are increasingly focusing on areas like explainable AI, which aims to make algorithms transparent and their decisions understandable to humans. This is crucial in sectors such as healthcare and finance, where accountability is paramount.
For instance, developments in reinforcement learning have opened up new avenues in robotics and automation. These advancements allow machines to learn through trial and error, much like humans do, enhancing their ability to make real-time decisions.
- Key impacts of advancements in AI algorithms include:
- Enhanced predictive capabilities
- Greater accuracy in decision-making
- Reduction in computational costs
The Impact of Quantum Computing
Quantum computing is still a nascent field, but its potential to revolutionize how we process data should not be underestimated. Traditional computers, while powerful, struggle with certain problems due to their linear processing nature. Quantum computers, on the other hand, leverage the principles of quantum mechanics to perform multiple calculations simultaneously.
This has profound implications for big data analytics and AI. For instance, data that would take classical computers years to process can be handled in mere minutes by quantum systems. As organizations collect colossal datasets, using quantum computing for data analysis could lead to breakthroughs in fields such as drug discovery and climate modeling.
An example of this is seen in how Googleâs quantum computer, Sycamore, achieved quantum supremacy by processing a specific calculation in 200 seconds, which would take a classical computer approximately 10,000 years.
The Growth of Edge Computing
With the proliferation of Internet of Things devices, edge computing is becoming increasingly relevant. Instead of sending all data to centralized servers for processing, edge computing allows data to be processed near the source of generation. This is a game changer, particularly for applications requiring real-time decision-making, such as autonomous vehicles and smart city infrastructures.
- Benefits of edge computing include:
- Reduced latency for time-sensitive decisions
- Decreased bandwidth usage, as less data is sent to the cloud
- Enhanced data privacy, since sensitive information can be processed locally rather than transmitted over networks
In summary, keeping an eye on these future trends in big data, AI, and machine learning is vital for professionals aiming to remain competitive in a fast-changing landscape. By embracing innovations such as advanced AI algorithms, quantum computing advancements, and edge computing growth, organizations can better position themselves for success in a data-driven world.
"The future is not something we enter. The future is something we create." - Leonard I. Sweet
Closure
In synthesizing the intricate threads woven throughout this article, it's clear that understanding the interplay of big data, artificial intelligence, and machine learning is paramount in todayâs digital age. This exploration has revealed how these technological forces not only coexist but bolster one another in transformative ways.
Summarizing Key Insights
To encapsulate the major themes, consider the following points:
- Interdependency of Technologies: Big data serves as the lifeblood for AI models, allowing them to learn and make predictions that are rooted in vast datasets.
- Machine Learning as an Accelerator: Through algorithms that unveil patterns hidden in data, machine learning elevates the efficiency and precision of AI applications across sectors such as healthcare and finance.
- Ethical Frameworks are Crucial: With great power comes great responsibility. The ethical implications of utilizing data and AI must be navigated with care to avert bias and protect privacy.
- Future Directions: As advancements like quantum computing and edge computing emerge, the synergy of these technologies will likely continue to reshape industries, redefining how we interact with the digital landscape.
The convergence of these fields heralds a future where enhanced decisions, faster innovations, and smarter systems are not just attainable but essential.
Looking Ahead
Looking toward the horizon, the trajectory for big data, AI, and machine learning is laden with potential and challenges. The following considerations stand important:
- Advancements in Algorithm Design: Future research and development will likely refine existing algorithms, enabling AI to process data in a more human-like fashion, enhancing decision-making significantly.
- Privacy Regulation Evolution: As data privacy concerns grow, so too will the regulatory landscape, resulting in more stringent laws. Organizations must be proactive in adapting their data practices to remain compliant.
- Real-time Data Processing: The push for faster data handling will drive innovations in edge computing, leading to applications in IoT environments that require instant data analysis and reactions.