Essential Knowledge for Mastering Machine Learning
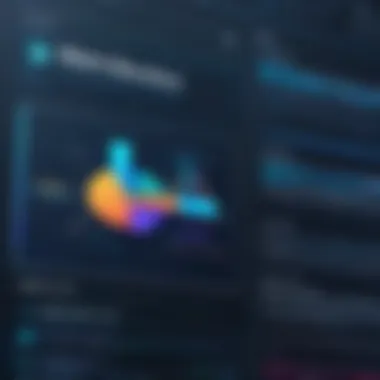
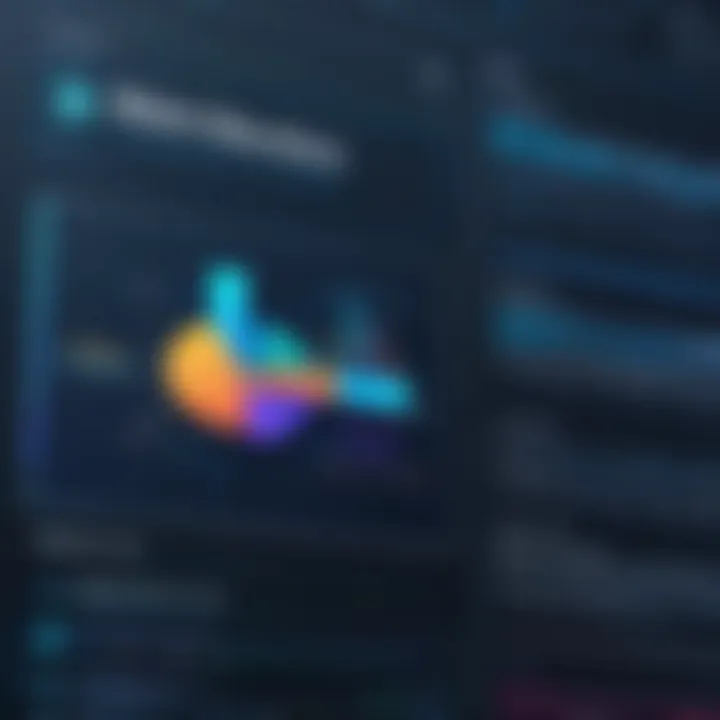
Intro
Machine learning is one of the most pivotal fields in technology today, yet its intricacies can often seem like an uncharted territory for many. Before one leaps into the captivating world of algorithms and data, there are several foundational stones that need laying. This article aims to unravel the essential prerequisites that anyone diving into machine learning should be well-versed in. From statistics to programming languages, these are the cornerstones that set the stage for effective engagement with machine learning.
By gaining insights into these essential skills and concepts, individuals can create a clearer pipeline towards successful machine learning projects. The journey begins with understanding coding challenges, not just as obstacles but as valuable learning experiences that sharpen one's problem-solving skills.
Coding Challenges
Engaging with coding challenges can foster significant growth in your programming prowess. It’s more than just writing lines of code; it helps frame your understanding of machine learning’s complex nature.
Weekly Coding Challenges
Participating in weekly coding challenges cultivates a habit of regular practice. Websites like HackerRank and LeetCode offer a plethora of problems specifically tailored to hone your skills. Often, these platforms provide not only a problem statement but also constraints and examples to clear up any ambiguity.
Problem Solutions and Explanations
Each problem you tackle often has a discussion section where solutions are explained. This is essential. It provides insights into different approaches, revealing a variety of tactics used to solve similar issues. Understanding how others reach a solution allows you to expand your own toolkit.
Tips and Strategies for Coding Challenges
When approaching coding challenges, remember:
- Break problems into smaller parts. When faced with a complex problem, dissecting it can make it more manageable.
- Practice consistently. Like any skill, regular exposure and usage improve comprehension.
- Don’t fear failure. Every wrongly answered question is an opportunity to learn and adapt.
Community Participation Highlights
One of the most powerful aspects of engaging with coding challenges is community interaction. Participating in forums on places like Reddit can expose you to varying perspectives and solutions you might not have considered.
"Collaboration often leads to breakthroughs that solitary efforts cannot achieve."
Being part of a community can provide motivation when the going gets tough, as well as offer mentoring opportunities.
Technology Trends
As machine learning continues to evolve, being aware of the technology trends surrounding it is critical. This knowledge can shape a robust understanding of where machine learning is heading and how to stay anchored in this fast-paced domain.
Latest Technological Innovations
Keeping abreast of innovations, such as advancements in deep learning frameworks like TensorFlow and PyTorch, can give you an edge. Understanding how these tools evolve aids in selecting the right ones for your specific needs.
Emerging Technologies to Watch
Keep an eye on the burgeoning domain of Natural Language Processing and how it is reshaping industries. Areas such as sentiment analysis and chatbots are only the tip of the iceberg.
Technology Impact on Society
Machine learning isn't just an isolated technical challenge; its applications affect numerous societal aspects, from healthcare to personalized marketing. Understanding these implications can enrich your perspective and bring more depth to your projects.
Expert Opinions and Analysis
Gleaning insights from industry professionals can provide invaluable understanding. Reading expert analyses helps you grasp practical applications of theoretical concepts. Keep an eye on respected platforms for blogs and articles to broaden your outlook on industry practices.
Coding Resources
Equipped with the right coding resources, anyone can sharpen their understanding and skills. From language guides to software reviews, the availability of quality materials cannot be overstated.
Programming Language Guides
Where to start? Python remains the go-to language for most machine learning projects due to its simplicity and rich libraries. Having solid language foundations is non-negotiable.
Tools and Software Reviews
Utilizing the right tools—like Jupyter for code exploration or Pandas for data manipulation—can streamline your workflow. Regularly reviewing these tools can keep your arsenal sharp.
Tutorials and How-To Articles
Step-by-step tutorials that break down complex concepts into digestible parts are invaluable. They aid in building a framework for learning, making everything less daunting than it might appear on first glance.
Online Learning Platforms Comparison
Platforms such as Coursera, edX, and Udacity offer structured learning paths tailored to machine learning. Having a clear understanding of what each offers can help you choose the right one for your learning style.
Computer Science Concepts
An effective grasp of certain computer science concepts is foundational for machine learning.
Algorithms and Data Structures Primers
Understanding core algorithms and data structures is crucial. A strong base allows you to implement and optimize machine learning algorithms more effectively.
Artificial Intelligence and Machine Learning Basics
Start with the fundamental concepts of AI that feed into machine learning. Familiarizing yourself with supervised and unsupervised learning sets a strong groundwork for further exploration.
Networking and Security Fundamentals
In the data-driven world, understanding networking and security principles create a backdrop for tackling common pitfalls. Securing data is paramount, especially in projects that deal with sensitive information.
Quantum Computing and Future Technologies
While it may sound futuristic, exposure to quantum computing concepts can set you apart. It’s the next frontier that could reshape machine learning as we know it.
Understanding Machine Learning
Machine learning is like the hidden engine propelling today's technological marvels. It's not just about crunching numbers; it’s about finding patterns and making informed decisions based on data. In this section, we’ll peel back the layers to reveal what machine learning really is and why it holds such significant sway over modern developments.
Definition and Scope
Machine learning can be defined as a subset of artificial intelligence that empowers systems to learn from data and improve their performance over time without being explicitly programmed. This iterative learning process allows machines to identify trends, recognize complex relationships, and adapt to new data inputs.
It's the difference between programming a computer to follow a set of instructions versus teaching it how to think based on past experiences. For instance, rather than coding rules for identifying spam emails, one could use a machine learning model trained on thousands of spam and non-spam emails. In its broadest sense, machine learning encompasses various techniques employed in data analysis, predictive modeling, and even robotics.
The scope of machine learning continues to broaden. The realm includes supervised learning, where the algorithm learns from labeled examples, and unsupervised learning, where it finds patterns in unlabeled data. Reinforcement learning also plays a vital role, often seen in applications like game AIs and autonomous systems. Each of these domains presents unique challenges and opportunities, which makes understanding this scope central to navigating the field effectively.
Importance in Modern Technology
In today’s world, where data reigns supreme, the relevance of machine learning is undeniable. Its applications permeate various industries, including finance, healthcare, tech, and even agriculture. Companies like Google and Amazon have harnessed machine learning for everything from recommending products to improving search algorithms.
Consider the impact on healthcare: machine learning helps in diagnosing diseases by analyzing medical imaging far beyond the capabilities of human doctors. It can process vast amounts of data, identifying risks with remarkable accuracy. In finance, it predicts market trends and automates trading with instant decisions based on real-time analysis.
Moreover, businesses leverage machine learning for optimized operations and personalized marketing strategies. The ability to tailor experiences based on individual behavior not only enhances user satisfaction but also significantly boosts revenue potential.
As we delve deeper into the prerequisites for engaging with machine learning, it becomes clear that understanding the foundational concepts of this technology is essential. With a robust grasp of its definition, scope, and importance, aspiring programmers, technology enthusiasts, and IT professionals can better navigate the complexities they will face throughout their journey into the world of machine learning.
"Machine learning is not the end—it's just the beginning of the journey into a smarter future."
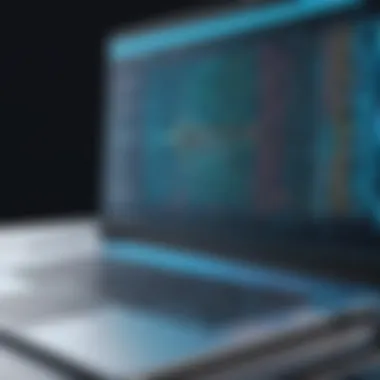
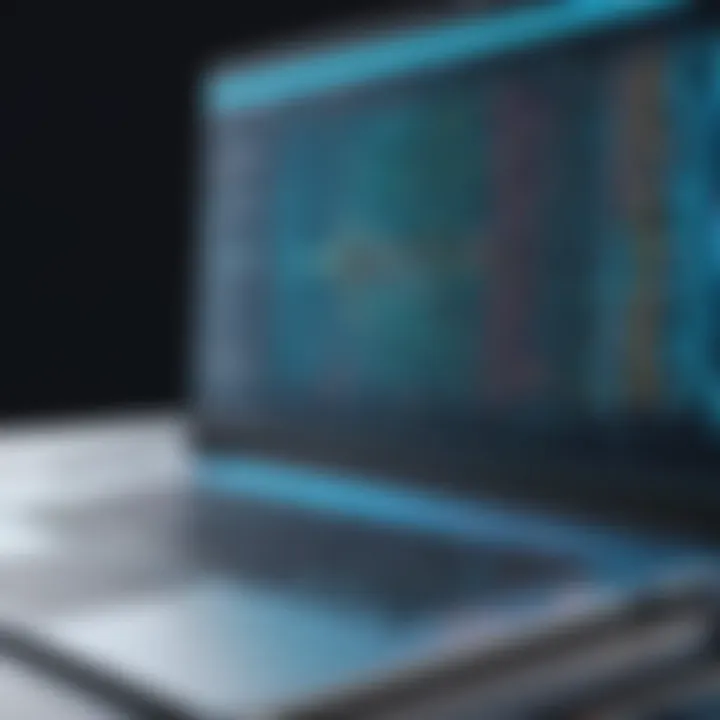
Statistical Knowledge
Statistical knowledge is more than just a collection of formulas and numbers; it's the beating heart of machine learning. As you gear up to dive into the world of algorithms and models, grasping the basics of statistics is crucial. This understanding not only helps in forming the backbone of your analyses but also enhances your intuition when evaluating various data-driven outcomes. Without a solid grounding in statistical principles, you risk shaping conclusions based on shaky assumptions, which can lead to erroneous predictions.
The benefits of statistical knowledge in machine learning can be summed up as follows:
- It enables you to summarize and describe large datasets effectively, helping in making informed decisions.
- Understanding statistical tests and measures aids in validating and selecting the right models.
- It fosters the ability to interpret outputs correctly, allowing for meaningful inferences based on data.
Descriptive Statistics
Descriptive statistics serve as the front door to understanding data. They provide a clear picture of what the data looks like without venturing too deep into inferential territory. With tools such as mean, median, and mode, you can spot trends and variability in your datasets.
For practitioners, it’s crucial to realize how skewness and kurtosis can paint a narrative about the data. For instance, if you observe a positive skew, that might indicate outliers or anomalies that can impact model training. Summarizing large data into a few key metrics not only helps in initial exploration but guides further modeling choices.
Key elements of descriptive statistics include:
- Measures of central tendency: mean, median, and mode give a sense of where the center of your data lies.
- Measures of spread: range, variance, and standard deviation showcase the distribution’s variability.
- Visualization techniques: histograms and box plots help in visually interpreting the underlying patterns.
"Descriptive statistics can turn mountains of data into nuggets of insight that are easier to digest."
Inferential Statistics
Inferential statistics are where the magic begins. This is the branch that allows you to make predictions and generalizations from a small sample to a broader population. Here, sampling techniques come into play, and familiarity with concepts like confidence intervals and hypothesis testing can greatly enrich your analytical arsenal.
Consider situations where you're testing the effectiveness of a new product. Using a sample to estimate how the entire population would respond not only saves time but also allows for informed decisions based on potential outcomes. In machine learning, this translates into how we assess model performance and reliability.
Crucial aspects of inferential statistics include:
- Hypothesis testing: understanding null and alternative hypotheses is fundamental in making data-supported conclusions.
- P-value: it helps determine the significance of your results, guiding whether to reject the null hypothesis.
- Confidence intervals: knowing how certain you are about your estimates can influence decision-making.
Probability Theory
Probability theory is the backbone upon which statistical inference stands. It’s about quantifying uncertainty and predicting outcomes based on various chances and likelihoods. A good grasp of probability is essential for understanding the behavior of random variables involved in various algorithms.
In the realm of machine learning, probability helps inform decisions when the outcomes are uncertain. For instance, models like Naive Bayes rely heavily on the concept of conditional probability.
Important components of probability theory include:
- Random variables: understanding how they can be manipulated is crucial for modeling.
- Distributions: familiarizing yourself with normal, binomial, and Poisson distributions can aid in recognizing patterns.
- Bayesian statistics: this approach offers a fresh perspective on probability and is gaining traction in many machine learning implementations.
By delving deeper into these statistical principles, you arm yourself with the knowledge needed to tackle the complex world of machine learning with confidence.
Programming Proficiency
Programming skills are not just a cherry on top in the field of machine learning; they are fundamental to navigating this complex landscape. The ability to code allows practitioners to create algorithms, manipulate data, and implement models that can learn from their inputs. Furthermore, programming proficiency helps streamline the entire workflow of a machine learning project, providing clear paths from data preparation to model deployment. Without a solid grasp of programming, even the most brilliant minds might find themselves lost in the vast ocean of machine learning tools and concepts.
Languages Commonly Used in Machine Learning
Python
Python stands out as the go-to language for many aspiring machine learning experts. One key aspect of Python is its simplicity and readability, allowing beginners to pick it up quicker than some other languages. Its extensive libraries tailored for scientific computing, like NumPy and pandas, make data manipulation a piece of cake.
As a versatile language, Python seamlessly integrates with various tools and technologies, creating a rich ecosystem for machine learning. A unique feature of Python is its supportive community and abundance of resources—think tutorials, forums, and libraries. However, it’s worth noting that Python can sometimes lag in performance speed, especially with large datasets. Still, its advantages often overshadow such drawbacks.
R
R is another language highly regarded within the machine learning realm, particularly among statisticians and data analysts. What sets R apart is its statistical prowess. Designed specifically for data analysis, it comes loaded with packages tailored for machine learning tasks. If you're diving into intensive statistical modeling or data visualization, R can provide tools that make those tasks more manageable and insightful.
A key characteristic of R is its ability to produce high-quality graphics right out of the box. However, it has a steeper learning curve compared to Python and can be less flexible for general-purpose programming—something to keep in mind if your goal is a broader tech skill set.
Java
Java may seem like an unconventional choice compared to Python and R, but it has its own merits in machine learning. Often chosen for its cross-platform capabilities, Java’s stability makes it an appealing option for production-level applications. What stands out about Java is its object-oriented nature, which can enhance the organization and management of codebases, especially important for larger projects.
A unique feature of Java is its strong performance in real-time applications, particularly when dealing with large amounts of data. However, the verbosity of Java can be a drawback, making it less accessible for beginners. The trade-off between performance and learning simplicity is a consideration worth pondering.
Understanding Libraries and Frameworks
NumPy
NumPy is crucial in the toolbox of anyone involved in machine learning, owing to its foundational role in numerical computing within Python. At its core, NumPy provides support for large, multi-dimensional arrays and matrices, along with a variety of mathematical functions to operate on these arrays. This library’s ability to perform element-wise operations efficiently offers an edge for data manipulation.
One key feature of NumPy is its speed. Written in C and able to leverage low-level optimizations, it offers significantly improved performance over regular Python lists. Its integration with other libraries in the Python ecosystem, such as matplotlib for visualization, makes it indispensable. Yet, for those completely new to programming, hands-on with NumPy might initially feel daunting.
Pandas
Pandas elevates the ease of data manipulation by introducing DataFrame objects, which bring structure to datasets often unorganized in raw formats. It allows users to perform operations like filtering, grouping, and aggregating data without navigating through a minefield of complex syntax. Recognized for its backbone role in cleaning and preparing data, Pandas is often a necessary step before modeling.
A remarkable feature of Pandas is its flexibility in handling both structured and unstructured data from various sources, including databases and file formats like CSV. However, performance may dip when handling extraordinarily large datasets, where more specialized tools may be required.
TensorFlow
TensorFlow, spearheaded by Google, is widely acknowledged for its capabilities in building deep learning models. Its architecture allows researchers and developers to construct complex neural networks with ease. One important aspect of TensorFlow is its support for both CPUs and GPUs, enabling faster computations—a massive advantage in the realm of machine learning where speed is often of the essence.
A unique feature of TensorFlow is using data flow graphs, which enhances the complexity of models built. This, however, can also be a double-edged sword; the learning curve may be steep for those not already familiar with concepts underlying graph-based computations. Yet, once surmounted, the potential for sophisticated modeling is immense.
Mathematical Foundations
In the realm of machine learning, one cannot emphasize enough the role that mathematical foundations play. These principles serve as a bedrock for understanding how algorithms function, guiding practitioners in their endeavors to develop efficient models. With a firm grasp on key mathematical concepts, programmers and researchers are better equipped to manipulate data, identify patterns, and improve the overall performance of their models.
Mathematics is not just an accessory; it’s an essential toolkit for anyone serious about diving into machine learning. For instance, linear algebra provides the language through which we express data and transformations, while calculus enables us to understand changes and optimize our models. Optimization techniques help us efficiently navigate cost functions to achieve desired outcomes. When these mathematical pillars come together, they unlock the complexity of machine learning, allowing for deeper insights and more effective solutions.
Linear Algebra
Linear algebra stands at the forefront of mathematical foundations. It deals with vectors, matrices, and linear transformations, playing a pivotal role in machine learning. Almost all machine learning algorithms fundamentally hinge on operations involving vectors and matrices. The essence of deep learning itself is often distilled down to understanding operations on multi-dimensional data structures.
Here's why linear algebra is critical:
- Data Representation: Every dataset can be represented as a matrix, where each row signifies an instance and each column signifies a feature. This representation is essential for processing and manipulating the data efficiently.
- Transformation and Projections: Algorithms often require transforming data into more useful forms. Linear transformations, such as rotation and scaling, are functions of matrices that can simplify complex datasets without losing essential information.
- Dimensionality Reduction: Techniques like Principal Component Analysis (PCA) utilize linear algebra to reduce the number of variables while retaining key features. This not only simplifies models but also speeds up computations.
"Linear algebra is the backbone of modern machine learning, making it easier to interpret and manipulate data at scale."
Calculus
Calculus follows closely behind linear algebra in its importance. It offers tools for understanding and modeling continuous change, crucial in optimizing machine learning models. By using derivatives and integrals, practitioners can determine how function outputs change concerning inputs, which is particularly useful in the tuning of models.
The benefits of calculus in machine learning include:
- Gradient Descent: This optimization algorithm relies on gradients to find the minimum of a cost function. Understanding how to calculate and apply gradients is fundamental for any practitioner aiming to minimize error.
- Activation Functions: Different activation functions, such as sigmoid or hyperbolic tangent, are formulated using calculus. Understanding their behaviors through their derivatives enables better choices in designing neural networks.
- Model Behavior Analysis: Calculus allows researchers to analyze how small changes in inputs affect outputs, offering insights that are crucial in refining model predictions.
Optimization Techniques
The heart of machine learning rests in optimization techniques. Once a model has been built using statistical methods, it requires fine-tuning to ensure the best performance. Optimization refers to the process of adjusting parameters for a desired output.
Here are key optimization concepts relevant to machine learning:
- Stochastic Optimization: Often used with large datasets, this technique randomly selects subsets for training instead of using the entire dataset. It enhances computation speed and generalization of the model without jeopardizing accuracy.
- Regularization: This technique adjusts weights to prevent overfitting by adding a penalty to the loss function. It ensures the model remains general rather than fitting noise in the training data.
- Adaptive Learning Rate Methods: Techniques such as Adam and RMSprop dynamically adjust learning rates during training, leading to more effective and faster convergence.
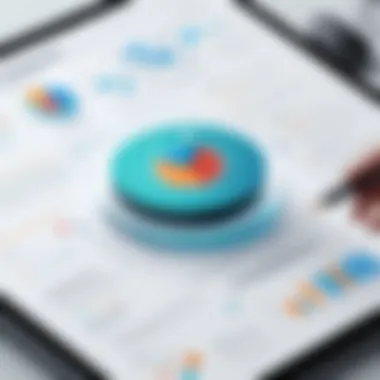
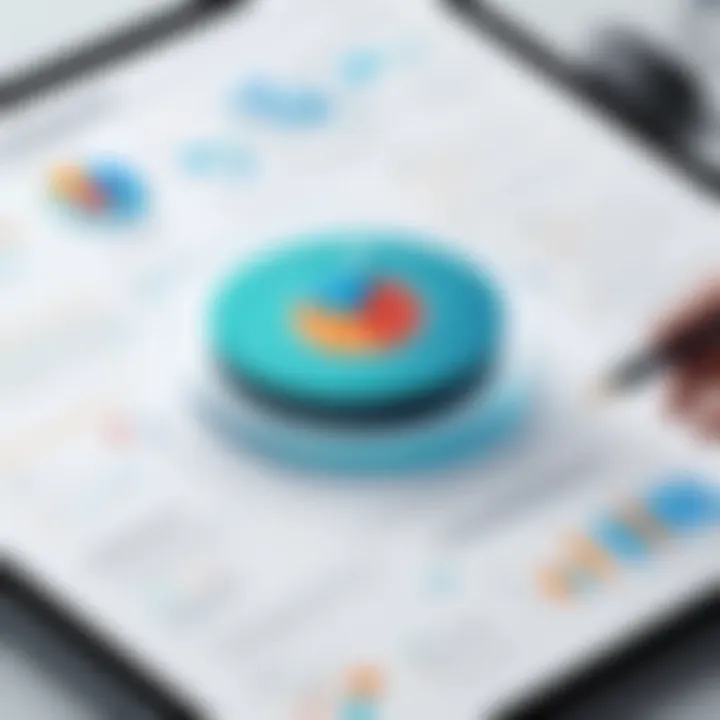
In summary, the mathematical foundations of machine learning are crucial. Understanding linear algebra, calculus, and optimization techniques lay the groundwork for all practitioners, enabling them to approach complex problems with confidence and clarity.
Data Handling Skills
Data handling skills form the backbone of successful machine learning implementations. Without the ability to properly manage and manipulate data, even the most advanced algorithms can falter. Mastery in this area ensures that data-driven insights are not just numbers and figures, but meaningful knowledge that can drive decision-making and innovation.
Being proficient in data handling means understanding how to gather relevant information, clean it effectively, and transform it to fit the specific needs of machine learning models. This process is not trivial; it requires a keen eye for detail and a commitment to quality. In an age where data is often referred to as the new oil, the ability to refine that oil into usable fuel becomes vital.
The benefits of robust data handling extend beyond mere technical aptitude. They include:
- Improved Model Performance: Quality data leads to better training outcomes.
- Time Efficiency: Well-organized and cleaned datasets save time during model training.
- Enhanced Decision Making: Accurate analyses provide valuable insights for stakeholders.
A solid grasp of data handling can significantly differentiate a novice from a seasoned professional in the field of machine learning.
Data Collection Methods
The first step in data handling is collection. Data can come from various sources, and understanding these can lead to better strategies for gathering the right quality and quantity of information.
Common methods include:
- APIs: Many platforms, such as Facebook and Twitter, provide APIs that allow developers to access their data programmatically. This can be a goldmine for data relevant to social trends or public opinions.
- Surveys and Questionnaires: Tailoring questions can yield specific insights tailored to research or business goals.
- Public Datasets: Organizations often release datasets on platforms like Kaggle or government databases, making them accessible for analysis.
Each of these methods has its own considerations, such as ethical concerns, geographical limitations, and the accuracy of the data collected.
Data Cleaning Techniques
Once data is collected, the next hurdle is cleaning it. Raw data is often messy and riddled with inconsistencies. Cleaning ensures that the information is reliable enough to inform model training.
Key techniques in this phase include:
- Handling Missing Values: It’s crucial to determine whether to fill in missing data, drop entries, or use models that can handle gaps naturally.
- Removing Duplicates: This is essential to avoid bias and skewed results during analysis.
- Standardizing Formats: Ensuring that all data entries conform to a consistent format can greatly enhance processing efficiency.
A well-cleaned dataset can save countless hours in later stages of analysis and model training.
Data Transformation Processes
Finally, transforming data into a suitable format for machine learning models is essential. This process can involve numerous techniques that convert raw data into structured datasets that algorithms can effectively interpret.
Common transformation methods include:
- Normalization: Adjusting values into a common scale, facilitating better comparison.
- Encoding Categorical Variables: Many algorithms function better with numerical data, hence transforming categories into numerical values is fundamental.
- Feature Engineering: This involves creating new variables from existing ones, potentially revealing deeper insights.
Determining the right set of transformations is a balancing act between complexity and performance. Each transformation should be evaluated based on its impact on model effectiveness.
In summary, effective data handling skills are crucial for any machine learning endeavor. Mastering the intricacies of data collection, cleaning, and transformation enables the utilization of machine learning techniques to their fullest potential.
Understanding Algorithms
In the realm of machine learning, grasping algorithms is akin to understand the engine of a car; without it, you may have the vehicle, but it won't go anywhere. Algorithms are the core of machine learning models and dictate how data is processed, insights extracted, and predictions made. A robust knowledge of algorithms empowers practitioners to select the right tool for the task at hand, enhancing model effectiveness and driving successful outcomes. Furthermore, understanding the intricacies of algorithms aids in troubleshooting, refining, and validating the models, fostering a comprehensive approach to machine learning endeavors.
Supervised Learning Algorithms
Regression Techniques
Regression techniques hold a prominent stature in the world of supervised learning. They are primarily used for predicting continuous outcomes based on input data features. For instance, if you wanted to forecast the price of a house based on its square footage, location, and number of rooms, regression analysis would be your go-to approach. Its power lies in the ability to model relationships and trends using mathematical functions. A key characteristic of regression techniques is their interpretability; practitioners can easily understand how each feature influences the prediction.
However, though they are popular, they come with caveats. One distinct aspect is the requirement of having a linear relationship between variables; if this assumption doesn't hold, predictions can be off the mark.
Advantages of regression techniques include:
- Simplicity: The models are straightforward to understand and implement.
- Versatility: They can be applied in various fields, such as economics, medicine, and real estate.
Disadvantages:
- Sensitive to Outliers: Anomalies in the data can significantly skew results.
- Assumption of Linearity: When relationships aren't linear, this method struggles.
Classification Techniques
Classification techniques are another pillar of supervised learning, tasked with categorizing input data into predefined classes or labels. A classic example is email filtering, where algorithms classify emails as spam or not based on various features like the subject line and sender. One of the primary characteristics of classification algorithms is their ability to handle categorical outcomes, which makes them essential in varied domains such as customer segmentation, image recognition, and medical diagnosis.
These techniques often include logistic regression, decision trees, and support vector machines, each with unique features. What sets classification methods apart is their performance measures, like precision and recall, which can effectively evaluate their accuracy in real-world applications.
Advantages include:
- Flexibility: They can manage both binary and multi-class outcomes.
- Performance Metrics: Offers measures like confusion matrices, which help to assess effectiveness.
However, disadvantages are present as well:
- Complexity: Some classification algorithms can be intricate and require deeper understanding to optimize.
- Overfitting: If not properly tuned, models can sometimes learn noise rather than the underlying pattern.
Unsupervised Learning Algorithms
Clustering Methods
Clustering methods represent a critical aspect of unsupervised learning, aiming to find inherent groupings within unlabeled data. Imagine trying to sort a basket of fruit; you group apples, bananas, and oranges without pre-defined categories. This is the essence of clustering. Popular techniques such as K-means and hierarchical clustering help in market segmentation, image compression, and even social network analysis.
What makes clustering special is its ability to uncover hidden patterns that might not be immediately clear. It allows for exploratory data analysis and can offer insights without the need for labeled data.
Benefits of clustering methods include:
- Insight Generation: Helps in discovering patterns within data.
- Adaptability: Useful in various domains from biology to marketing.
On the downside:
- Interpretation Difficulty: Results can be subjective and often require some domain expertise to make sense.
- Scalability Issues: Some algorithms struggle with very large datasets.
Dimensionality Reduction
Dimensionality reduction is a process that simplifies complex datasets while retaining their essential features. Imagine you have a very detailed photo but want a smaller version that still looks good; that's dimensionality reduction in action. Techniques like Principal Component Analysis (PCA) eliminate redundancy by reducing the number of variables in a dataset but capturing most of the information.
This is particularly useful when working with high-dimensional data, where noise and computationally expensive algorithms can hinder performance. A crucial characteristic of dimensionality reduction is its ability to facilitate visualization; simplifying data allows analysts to see trends and patterns that may not be as pronounced in multi-dimensional space.
Advantages include:
- Improved Efficiency: Shapes the data landscape, making algorithms faster.
- Noise Reduction: Helps in filtering out irrelevant features that don’t contribute to model performance.
Conversely, disadvantages can arise:
- Loss of Information: In the pursuit of simpler data, some vital details may be lost.
- Complexity in Interpretation: The new features may not be as interpretable as the original data.
Reinforcement Learning Concepts
Reinforcement learning stands on its own as it mimics the way humans learn from interactions with their environment. Here an agent makes decisions, observes outcomes, and, importantly, learns from consequences. This dynamic feedback loop allows for continual adaptation and improvement, making it exceptionally useful for complex decision-making tasks, like game playing or robotic controls.
The key characteristic here is the goal-oriented aspect: actions taken ultimately lead to rewards or penalties, fostering a kind of learning that can yield remarkable results over time. Understanding reinforcement learning concepts is pivotal, especially as industries lean more toward automation and intelligent systems. It presents both challenges and immense opportunities for growth and learning in the field.
Feature Engineering
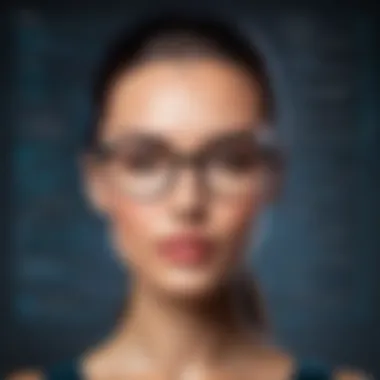
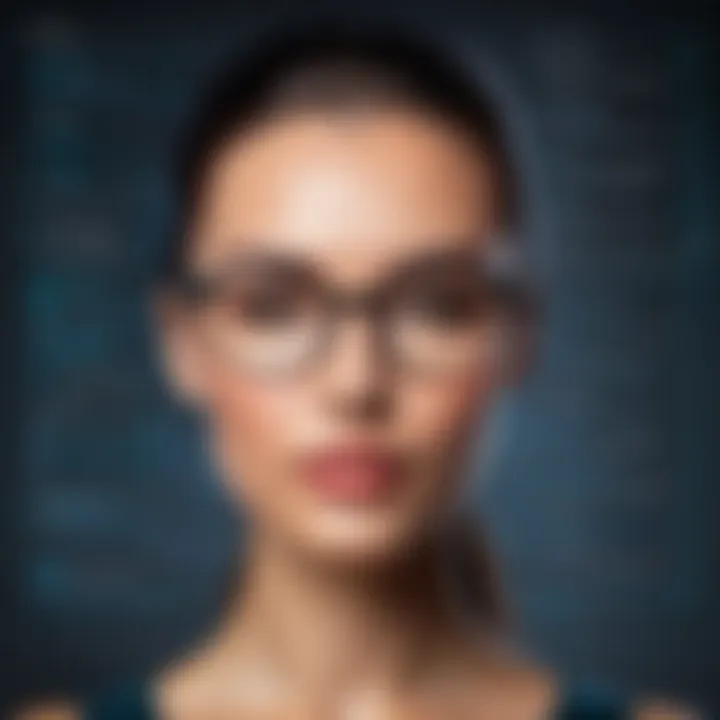
In the realm of machine learning, feature engineering stands as a cornerstone that significantly influences the performance of models. It is the process of selecting, modifying, or creating features that make your data more suitable for a machine learning algorithm. This process can often determine whether a model flounders or flourishes.
The importance of feature engineering can’t be overstated. A well-crafted feature set provides critical insights and allows algorithms to learn effectively. Machine learning models, after all, work primarily with the input they receive; thus, the right features can mean the difference between a mediocre performance and a model that excels.
Importance of Feature Selection
Choosing the right features is essential because it helps to reduce complexity and improve model accuracy. Often, more isn’t better when it comes to data; irrelevant or noisy features can dilute the signal, causing models to underperform. It’s like trying to listen to a beautiful melody drowned out by unnecessary background noise. Therefore, effective feature selection not only enhances predictive power but also aids in reducing overfitting.
"A simple model with strong features often outperforms a complex model with weak features."
When considering feature selection, a few methods can streamline the process:
- Filter Methods: These involve statistical tests to choose the features that have the strongest relationship with the output variable.
- Wrapper Methods: Here, features are selected by evaluating a model's performance and iterating through different combinations of features.
- Embedded Methods: These incorporate feature selection into the model training process, where algorithms themselves decide on feature importance as they build the model.
The selection should be guided by domain knowledge as well. Knowing which features might have more significance lets you make decisions that enhance model performance rather than relying solely on automated processes.
Techniques for Feature Extraction
Once the relevant features are identified, you may still need to transform them into a format that can be effectively used by machine learning algorithms. This is where feature extraction comes into play. It involves creating new features by combining or transforming existing ones to capture the underlying patterns in the data better.
There are several techniques for feature extraction you can consider:
- Principal Component Analysis (PCA): This technique reduces the dimensionality of data while preserving as much variability as possible. PCA creates new uncorrelated features from the original data, which can help in simplifying models.
- t-Distributed Stochastic Neighbor Embedding (t-SNE): Primarily used for visualizing high-dimensional datasets, t-SNE converts similarities into joint probabilities, aiding in understanding complex data structures visually.
- Feature Hashing: This is a fast and memory-efficient way to transform arbitrary features by using hash functions. It works well in natural language processing tasks and with large datasets.
Implementing these techniques can streamline a dataset and hone in on the features that truly matter, giving machine learning constructs a clearer path toward achieving their goals.
In summary, feature engineering is integral to the machine learning workflow. It not only prepares your data but also allows algorithms to perform more effectively, ultimately enhancing the insights derived from the data while tackling specific challenges with precision.
Model Evaluation
Model evaluation is a cornerstone in the realm of machine learning, serving as the litmus test to determine how well a model performs prior to deployment. This evaluation is crucial because it not only validates the model’s predictive capability but also helps identify areas that require refinement or adjustment. Without effective evaluation metrics, one could be entangled in a maze of uncertainty about a model's actual utility.
When machine learning models are trained, they learn patterns based on the data they ingest. However, this training needs careful examination to make sure the model generalizes well to unseen data. Here’s why this topic deserves careful consideration:
- Mitigate Overfitting: One of the most pressing concerns in model training is overfitting, where a model performs exceptionally well on training data but flounders on unseen data. Evaluating how a model behaves on distinct datasets helps in spotting this flaw early.
- Optimize Performance: Through methodical evaluation, practitioners can pinpoint which algorithms yield the best results for specific datasets, thereby aiding in fine-tuning and enhancing overall performance.
- Enable Informed Decisions: Insight gained from evaluations empowers data scientists and stakeholders to make informed choices about deploying models in real-world applications.
In this section, we will delve into the significance of differentiating between training and testing data, as well as the integral role of performance metrics.
Training vs. Testing Data
The distinction between training data and testing data is one that can’t be overstated. Training data is the bedrock upon which a model learns. It consists of data points the model uses to learn its patterns, correlations, and relationships. On the flip side, testing data serves as a proxy for real-world data, providing a means to evaluate a model's predictive capacity without bias.
Here are two primary purposes for separating these datasets:
- Avoiding Bias: Utilizing the same dataset for both training and testing will lead to skewed results, making a model look better than it actually is.
- Assessing Real-World Performance: Testing data allows for a realistic evaluation of a model's efficacy, independent of the patterns it learned from the training set.
Performance Metrics
Performance metrics are vital for assessing models. They give insight into how well a model classifies inputs and ensures that the investment in model training translates into valuable outputs. The following subsections will elaborate on three key performance metrics: accuracy, precision, and recall.
Accuracy
Accuracy is often the go-to measure for evaluating a model, calculated as the ratio of correctly predicted instances to the total number of instances. While it provides a quick snapshot of overall performance, there are nuances to its use.
- Key Characteristic: Its ability to provide an easy-to-understand statistic makes it widely appreciated among practitioners.
- Popular Choice: Many stakeholders lean on accuracy due to its simplicity, particularly in balanced datasets where classes are distributed evenly.
- Unique Feature: One of its advantages is its straightforwardness; however, it has a significant drawback—the risk of masking poor performance in imbalanced datasets. If one class is dominant, accuracy can be misleading.
Precision
Precision assesses the number of true positive predictions relative to the total predicted positives. It’s particularly valuable when the cost of false positives is high, as in fraud detection or medical diagnoses.
- Key Characteristic: High precision indicates a low rate of false positives, which is critical in fields like healthcare.
- Considered Beneficial: Precision may carry more weight than accuracy when dealing with imbalanced classes, ensuring that the model is reliable in predicting the positive class when it does.
- Unique Aspect: While it focuses on positive predictions, it does not account for actual positive cases, which means using precision alone might lead to incomplete assessments of a model's effectiveness.
Recall
Recall, often termed true positive rate, examines how many true positives were correctly identified out of all actual positives. This metric gains importance in scenarios where missing a positive case is detrimental, such as in oncology.
- Key Characteristic: It emphasizes sensitivity, specifically in identifying positive instances, and is pivotal in applications where failing to detect a true positive can lead to severe consequences.
- Well-Chosen Metric: Using recall is beneficial for models with a focus on ensuring all possible positive instances are identified, even at the expense of getting some false positives.
- Unique Feature: While it casts a broad net to catch as many positives as possible, this can sometimes dilute the precision, leading to a balance concern.
Domain Knowledge
In the realm of machine learning, having domain knowledge is like having the key to a treasure chest. It is not only valuable but also an essential component that influences the success of any project. When you understand the specific industry or field you are working in, you're better equipped to tackle the challenges that arise. This understanding allows practitioners to frame problems accurately and devise effective solutions tailored to the unique needs of that domain.
The significance of domain knowledge can be broken down into several key elements:
- Improved Problem Identification: Recognizing what problems are truly worth solving in a given field is half the battle. Domain experts can pinpoint critical issues that may go unnoticed by others, leading to insightful machine learning applications. For instance, in healthcare, understanding symptoms and treatment protocols aids in developing predictive models for patient outcomes.
- Data Relevance and Quality: Whether it's finance, agriculture, or social media, each domain has specific types of data that are more relevant for analysis. Experts can guide efforts to collate and cleanse datasets so that they reflect real-world situations accurately. Misleading data can result in erroneous conclusions, so having domain guidance is crucial.
- Informed Feature Selection: Knowing what features to select can drastically alter model outcomes. For example, in retail, understanding customer behavior can guide feature engineering to focus on transactions, seasonality, and demographics that genuinely impact sales.
"Informed decisions arise from recognizing the intricacies of a field. The more you know, the better your expertise in navigating machine learning."
- Contextual Interpretation of Results: Data-driven insights require an interpretation that's grounded in knowledge of the domain. Metrics gained from analysis must be viewed in context; otherwise, they may lead to misguided actions. For example, a spike in user engagement in a tech application could mean success, but if it’s driven by a temporary marketing gimmick, it may not be sustainable.
Having emphasized that, let's dive deeper into the specific applications and challenges in understanding various domains of knowledge, particularly relating to machine learning.
Industry-Specific Applications
Each industry presents unique opportunities and challenges for machine learning applications. Tailoring approaches to meet these specifics can lead to transformative impacts.
- Healthcare: Machine learning algorithms are used to predict disease outbreaks, recommend treatments, and personalize patient care. Professionals in health must comprehend medical terminologies, patient histories, and the intricacies that differentiate one ailment from another.
- Finance: Here, models predict stock movements, assess credit risk, and detect fraudulent activities. Understanding financial regulations and market behavior can essentially improve the effectiveness of machine learning models. Knowledge of economic indicators like interest rates or inflation rates is pivotal.
- Manufacturing: Applying machine learning for predictive maintenance or optimizing supply chains requires a grasp of the manufacturing processes, machinery, and operational challenges. Engineers must work closely with data scientists to identify the factors affecting production efficiency.
- Retail: Customer buying patterns can be enhanced through recommendation systems that benefit from thorough understanding of market trends and the specific demographics of a target customer base.
By embedding domain knowledge into these applications, solutions become more relevant, impactful, and sustainable; ensuring a higher success rate in machine learning endeavors.
Understanding Business Challenges
For machine learning projects to truly succeed, it's imperative to fathom the business landscape in which they operate. This means identifying obstacles that the business faces and translating them into theoretical machine learning queries. It requires more than just technical skills; it’s about understanding the broader ecosystem in which these technologies will be incorporated.
- Identifying Key Performance Indicators (KPIs): Knowing which metrics are vital for success in a business can steer how one approaches machine learning solutions. For instance, a retail firm might focus on conversion rates, while a cybersecurity company might prioritize incident response times.
- Resource Allocation: Deciding where to invest time and capital is crucial. This can involve determining which problems are manageable and ripe for machine learning application versus those that may be too complex or expensive to solve.
- Risk Management: Every business decision carries some risk. Understanding potential pitfalls in applying machine learning strategies allows for better planning and mitigation strategies.
In sum, domain knowledge isn't merely a nice-to-have; it is an essential ingredient for making machine learning successful. Without it, even the most sophisticated algorithms may falter when faced with real-world complexities.
Continual Learning
In the ever-evolving landscape of technology, especially in machine learning, continual learning has become a critical pillar that professionals cannot afford to overlook. It is not merely a trend; it's a necessity born from rapid advancements and the expanding complexity of the tools and methodologies used in the field. The pace at which algorithms improve and datasets grow signifies that the learning never really stops after a foundational knowledge has been acquired.
Continual learning emphasizes a commitment to staying informed and relevant. For those venturing into machine learning, grasping new techniques or technologies is vital. The shift from traditional programming to a data-centric approach highlights the pressing need for ongoing education. Those equipped with a habit of continual learning will find themselves better positioned to adapt to changes in the field, whether they pertain to new libraries, programming paradigms, or architectural innovations.
Staying Updated with Advancements
To stay at the forefront of machine learning, professionals need to continuously update their knowledge base. This means keeping an eye on recent papers, conferences, and advancements emerging from research labs and industry. Reading white papers and technical blogs, digesting research articles via resources like arXiv or utilizing platforms such as Medium can offer insights straight from the innovators themselves.
Furthermore, many online courses and webinars regularly emerge, addressing cutting-edge developments within AI and machine learning. Engaging in platforms like Coursera or edX enables enthusiasts to learn directly from institutions renowned in the tech world. In addition, popular forums like Reddit serve as vibrant communities where practitioners exchange knowledge and resource recommendations.
This makes it essential for each machine learning practitioner to cultivate a habit of habitual reading and active participation in lifelong learning. By doing so, they can not only polish their existing skills but also sidestep obsolescence in a fast-paced, dynamic industry.
Engaging with the Community
Engagement with fellow learners and professionals is another potent strategy in the realm of continual learning. The machine learning community is robust and comprises diverse individuals, from enthusiastic beginners to seasoned experts. Interaction within this sphere can often lead to discovering invaluable resources and networking opportunities.
Participating in meetups, hackathons, and tech conferences can offer real-world exposure and collaboration that online learning often lacks. Platforms like Facebook can also have groups specifically for machine learning practitioners where knowledge is shared freely.
Additionally, contributing to open-source projects on GitHub can significantly bolster one’s skills. This not only facilitates learning through practical experience but also helps in forming connections with other contributors who share similar interests.
In sum, engaging with the community—whether by sharing, teaching, or simply collaborating on a project—is instrumental in reinforcing one's knowledge and passing it forward, maintaining a cycle of mutual growth. As the saying goes: “If you want to go fast, go alone; if you want to go far, go together.” The importance of collaboration, in this context, cannot be overstated.
“Innovation thrives on collaboration.”