Monetizing Machine Learning: Strategies for Income Generation
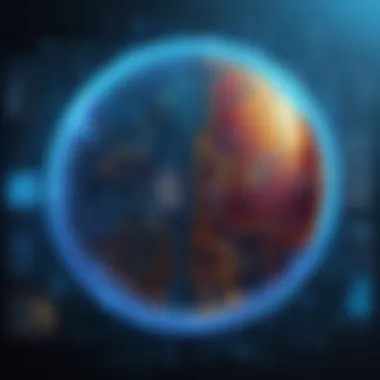
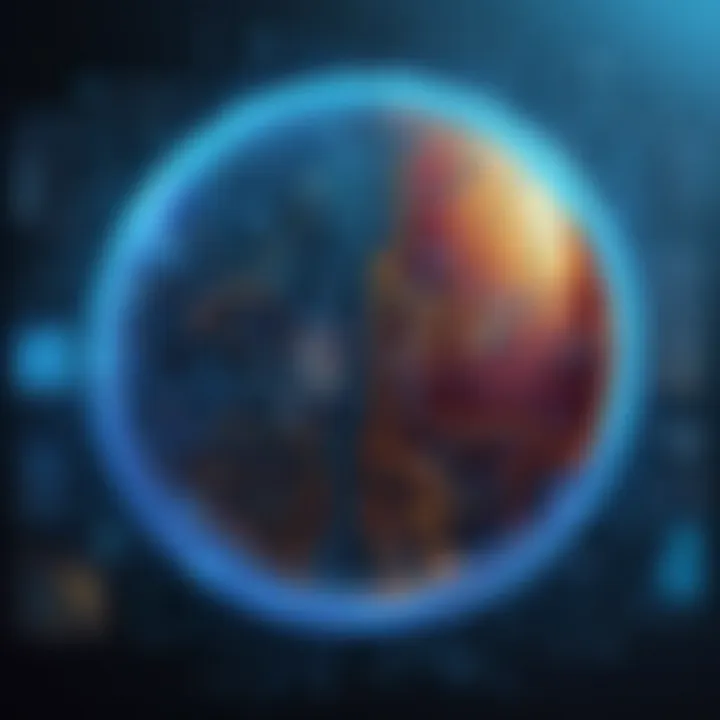
Intro
In today’s rapidly evolving landscape, machine learning stands as a cornerstone of innovation and problem-solving across numerous industries. The fascination with machine learning technologies is not just confined to academia; it's a goldmine for those keen to turn their skills into profitable ventures. The drive to monetizing machine learning opens up various doors, from freelance gigs to high-paying jobs in specialized areas.
Grasping the market's pulse is crucial. What do businesses need? What solutions can machine learning provide? Awareness of these questions can imagine a wealth of opportunities that lie ahead. This guide aims to illuminate practical strategies for harnessing machine learning capabilities and transforming them into financial gain.
With the right skills and approach, aspiring programmers, tech enthusiasts, and professionals can navigate this terrain and reap substantial rewards. The following sections will delve into significant trends, valuable resources, and compelling coding challenges that enhance both expertise and career prospects.
Prelims to Machine Learning
In today's fast-paced tech world, understanding machine learning is not just a luxury; it’s a necessity. As industries increasingly rely on data-driven decisions, having machine learning knowledge opens numerous doors for individuals looking to diversify their income sources. It’s like having a ticket to the big leagues of technology. This section sets the groundwork for monetizing these crucial skills, providing a framework that aspiring programmers, technology enthusiasts, and IT professionals can build upon.
What is Machine Learning?
At its core, machine learning involves algorithms that enable computers to learn from and make predictions based on data. It's all about teaching machines to recognize patterns and adjust their actions based on insights collected from vast amounts of information. Essentially, it’s like training a dog; with enough practice and positive reinforcement, the dog learns to sit, stay, or fetch—a useful adaptation over time.
Here’s a simpler breakdown:
- Supervised Learning: The algorithm learns from labeled data, meaning the input is paired with the correct output.
- Unsupervised Learning: Here, the algorithm tries to find hidden patterns or intrinsic structures in input data without pre-existing labels.
- Reinforcement Learning: This involves algorithms that learn optimal actions through trial and error, often used in gaming or robotics.
By understanding these different types, one can better appreciate the vast applications machine learning has to offer, shaping everything from online recommendations to advanced robotics.
Applications in Various Industries
Machine learning is a jack-of-all-trades, influencing multiple sectors in significant ways. Think about it:
- Healthcare: Machine learning can help in predicting disease outbreaks or identifying effective treatment plans by analyzing patient data.
- Finance: Algorithms used in fraud detection and risk assessment analyze patterns and anomalies in financial transactions, saving companies millions.
- Retail: Personalization engines enhance customer experiences by suggesting products based on previous purchases, boosting sales.
- Transportation: Self-driving cars rely heavily on machine learning to process data from sensors and navigate safely.
Each industry is essentially sitting on a goldmine, ready to be tapped for financial gain with the right machine learning strategies in play. By comprehensively understanding how this technology impacts diverse fields, individuals can strategically position themselves to profit from their skills while addressing real-world challenges.
"The only way to do great work is to love what you do." – Whether it's programming or data analysis, fueling passion in your pursuit will lead to greater success.
To wrap it up, wrapping your head around what machine learning is and its multifaceted applications prepares you well for the journey ahead in monetizing your skills. Understanding these fundamental aspects is step one; the potential for financial gain lies ahead.
Understanding the Financial Potential
In today's fast-paced environment, it's crucial to grasp the financial opportunities that come with machine learning technologies. The value it brings isn't just theoretical; it transforms businesses across various sectors by improving efficiencies and driving innovation. Those who understand this potential can better position themselves to capitalize on their skills.
Machine learning can no longer be viewed as just an academic interest or a buzzword. It’s a powerhouse shaping industries, leading to significant market demand. Recognizing the financial potential means not only identifying current opportunities but also staying ahead of the curve for future developments. Ignoring this aspect could leave money on the table, as companies actively seek individuals who can provide data-driven solutions.
Some key benefits of understanding this financial landscape include:
- Informed Decision-Making: Knowing market demands helps professionals tailor their skills and offerings.
- Competitive Edge: Those who grasp the economic trends can better position themselves against peers.
- Long-Term Planning: A clear view of the financial potential allows for strategic investments in further education and skill development.
"The future belongs to those who believe in the beauty of their machine-learning ideas."
Market Demand for Machine Learning
The current landscape is rife with opportunities for machine learning professionals. Companies across tech, finance, healthcare, and retail are salivating over the efficiency that AI and machine learning bring to the table. As more businesses begin to harness these technologies, the demand for skilled practitioners is only poised to grow.
Reports suggest that the market for machine learning could reach billion-dollar valuations in the years to come. Factors contributing to this surge in demand include:
- Data Explosion: With the vast amounts of data generated daily, businesses need specialists to analyze and make sense of this information.
- Technological Advancements: Continuous improvements in algorithms and computing power mean that machine learning applications are becoming more accessible.
- Industry Adoption: From predictive maintenance in manufacturing to personalized experiences in e-commerce, almost every field is integrating ML solutions.
Being aware of such demand positions you to seize various opportunities, whether it be through freelancing or accepting full-time roles.
Current Trends and Future Projections
With technology evolving at a dizzying pace, staying informed on trends is non-negotiable. Among the most notable trends affecting the machine learning space are:
- Automated Machine Learning (AutoML): This trend is democratizing machine learning, allowing individuals without a deep programming background to apply its principles effectively. As more tools become user-friendly, the demand for teaching and support roles is likely to expand.
- Real-Time Data Processing: Organizations are increasingly looking for ways to process data on the fly. This focus is driving growth in real-time analytics, which could open new avenues for businesses to monetize their data.
- Ethical AI Development: Companies are more vigilant about bias and ethical considerations in ML models. Professionals who understand these challenges and can effectively address them will be in high demand.
Looking forward, the future remains bright for machine learning revenue opportunities. Projections indicate robust growth, with estimates suggesting a doubling market size within just a few years. Those prepared to ride this wave will find themselves in a favorable position, both in terms of career satisfaction and financial gains.
Diverse Avenues to Generate Income
The potential for machine learning to enhance income opportunities is substantial. As businesses increasingly rely on data-driven decisions, the demand for skilled individuals who can harness machine learning techniques continues to grow. Understanding the various ways to monetize machine learning knowledge is crucial for anyone looking to benefit financially in this domain. By offering diverse pathways, individuals can tailor their approaches based on their skills and interests, providing a sense of direction as they navigate the evolving tech landscape.
Freelance Opportunities
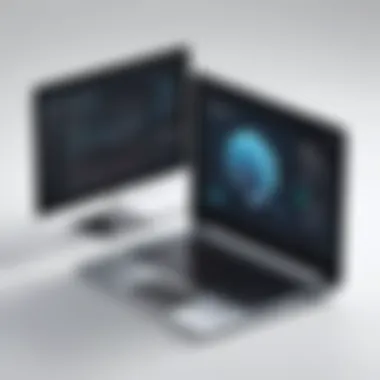
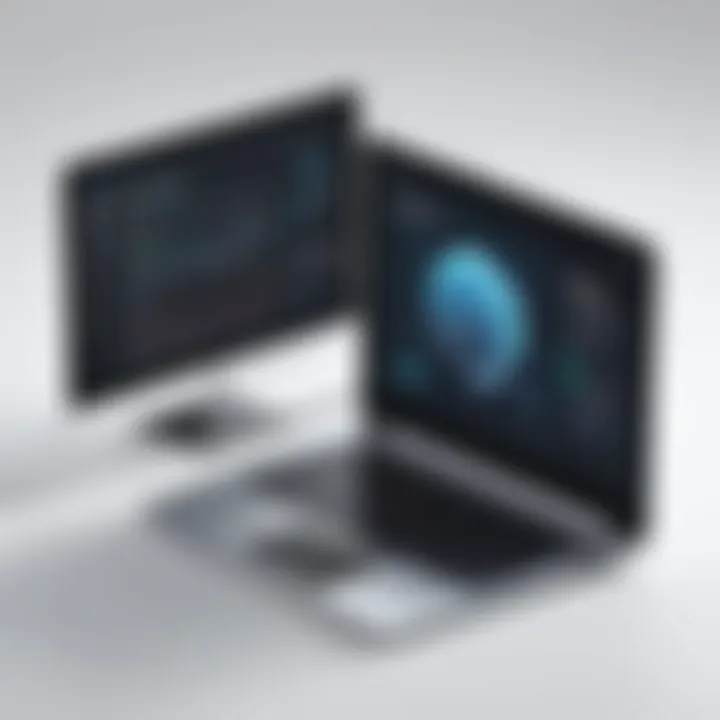
In recent years, freelancing has emerged as an appealing option for many in the tech industry. Freelancers have the flexibility to choose projects that align with their expertise while working on their own schedule. For machine learning practitioners, this could mean undertaking tasks such as developing predictive models for businesses or analyzing datasets to uncover trends.
Some lucrative freelance platforms include:
- Upwork: Reliable place to find clients looking for machine learning solutions.
- Toptal: A platform connecting top freelancers with elite companies.
- Guru: Wider range with competitive rates.
It’s essential to have a strong portfolio to showcase your projects. Consider contributing to platforms like GitHub with some unique projects. It’ll not only enhance your profile but also attract potential clients.
Consulting Services
Consulting can be a profitable avenue to explore. As organizations increasingly emphasize data-driven strategies, they often seek expertise to boost their machine learning capabilities. In this role, consultants can provide tailored insights, helping businesses to implement models and frameworks that fit their needs.
Having extensive knowledge in specific industries can provide a competitive edge. For instance, if you’re well-versed in healthcare or finance, emphasizing that expertise during consultations can create a niche market for yourself. Proper marketing of your consulting services is crucial; leveraging platforms like LinkedIn can be beneficial for outreach.
Full-Time Employment in Tech Firms
Joining a tech firm as a full-time employee offers stability along with the opportunity to work on large-scale projects. Many companies seek machine learning engineers, data scientists, and analysts, offering competitive salaries and benefits.
- Job roles to consider:
- Machine Learning Engineer
- Data Scientist
- Data Engineer
It's advisable to keep an eye on companies that are investing heavily in AI. They not only pay well but can also provide a rich learning experience. Participating in internships or boot camps related to your desired tech firm can prove advantageous when applying for full-time positions.
Developing Machine Learning Products
Creating your own machine learning product can be a game-changer. Whether it’s an app that utilizes predictive analytics or a tool for automating data processing, the sky's the limit. The key is to identify a gap in the market where your skills can provide a solution.
Here are some steps to start:
- Identify a problem that can be solved through machine learning.
- Validate your idea through market research.
- Develop a minimal viable product (MVP) to test.
- Gather feedback and iterate based on user responses.
This approach can lead to long-term revenue through subscriptions or sales, making it a worthwhile investment.
"The best ideas often come from the simplest of problems."
In wrapping this section, the diverse avenues of income generation within the machine learning sphere not only enhance financial prospects but also foster growth in knowledge and skills. Integrating these methods into a career strategy can profoundly impact both personal and professional development.
Essential Skills for Profitability
To successfully monetize machine learning proficiencies, it's essential for individuals to cultivate a robust skill set. This not only enhances one's capabilities but also provides a competitive edge in a field that is continuously evolving. Without the right skills, even the most promising opportunities may slip through one's fingers.
A focus on foundational knowledge, practical tools, and techniques for handling data will significantly increase one's prospects for profitability. Let’s break down these essential components further:
Foundational Knowledge in Statistics and Mathematics
Statistics and mathematics serve as the backbone of machine learning. This isn’t just a matter of familiarizing oneself with formulas. It’s about developing a deep understanding of concepts like probability distributions, inferential statistics, and hypothesis testing. A strong grounding will help decipher complex algorithms and validate models effectively.
- Why It Matters: Most machine learning algorithms, whether supervised or unsupervised, rely on statistical principles. Grasping these principles allows you to fine-tune models and interpret results accurately.
- Key Areas to Focus On: Explore topics like regression analysis, linear algebra, and calculus. Understanding how these concepts interlink will aid in practical applications.
Statistics won't just help with understanding models; it will also drive better decision-making in business contexts. If you can grasp data variability and trends, you can offer more valuable insights to clients and organizations.
Programming Languages and Tools Proficiency
Proficiency in programming is a non-negotiable skill in the machine learning landscape. Languages like Python and R have emerged as leading tools, largely due to their extensive libraries tailored for machine learning.
- Python Libraries: Don't overlook crucial libraries such as TensorFlow, Scikit-learn, and Keras. These libraries streamline the entire process, from data manipulation to modeling.
- R's Advantages: R may be preferred for statistical analysis and has strong graphical capabilities. Knowing both can make you versatile.
Proficiency in programming goes beyond writing code. It involves being able to translate business requirements into machine learning solutions. To achieve this, familiarize yourself with integrated development environments like Jupyter Notebook and RStudio.
Data Handling and Processing Techniques
Data is often referred to as the new oil, but just like oil, it needs to be refined before it can be useful. Mastering techniques for data collection, cleaning, and preprocessing is crucial for any machine learning endeavor.
- Data Cleaning: This involves identifying inaccuracies in data and deciding how to handle missing values. Techniques like imputation or deletion are just a few strategies you might employ.
- Processing Techniques: Understanding how to manipulate data using frameworks like Pandas or NumPy in Python is critical. This will aid not just in handling datasets but also in enhancing overall model performance.
When working with large amounts of data, knowledge of tools such as Hadoop or Spark can be highly beneficial. This allows for processing big data efficiently, enabling you to provide timely insights that could lead to significant innovations.
In summation, developing the essential skills outlined above sets the stage for a successful career in machine learning. Whether you’re looking to freelance, consult, or find a full-time position, these foundational elements are integral to making a meaningful impact in the field and ensuring your financial success.
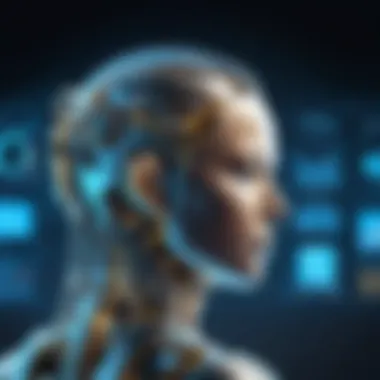
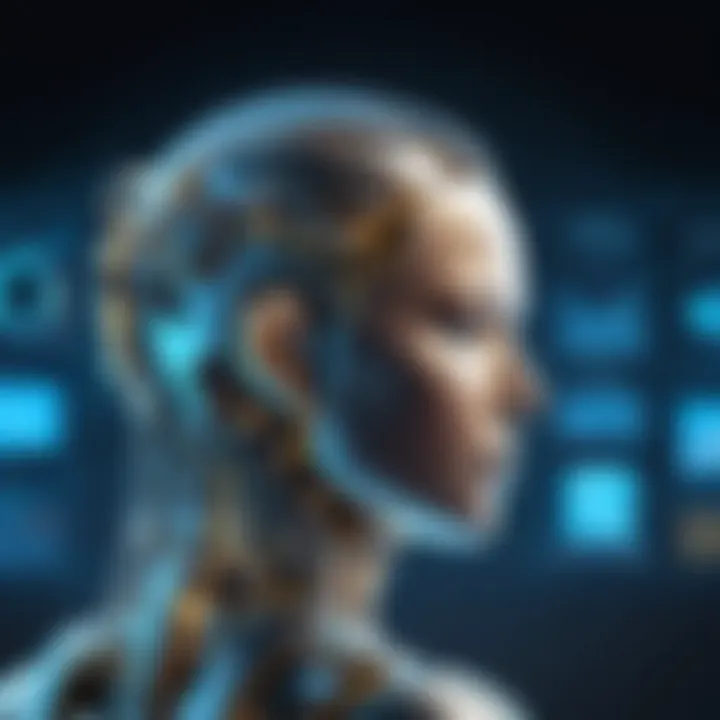
"Investing time into these skills today will pay off in dividends tomorrow."
By cultivating a thorough understanding of statistics and mathematics, honing programming skills with the right tools, and mastering data handling techniques, individuals can maximize their earning potential in the machine learning market.
Harnessing Online Platforms
In the landscape of machine learning, finding the right avenues to leverage one's skills can be the golden ticket to financial success. Online platforms serve as pivotal enablers of this journey. They allow machine learning aficionados—from fresh college graduates to seasoned professionals—to connect, share knowledge, and monetarily benefit from their expertise. This section takes a closer look at the distinct opportunities that arise from harnessing these platforms, emphasizing the necessity, advantages, and critical considerations involved.
Offering Courses and Workshops
Creating and offering courses or workshops is one of the most effective ways to convert knowledge into income. With the expanding interest in machine learning, many learners are eager to gain practical skills in this domain. Whether it's through platforms like Coursera, Udemy, or even your own website, designing a course that speaks to your unique experience can be particularly lucrative.
- Audience Targeting: Understanding your audience is key. Are you teaching beginners who are just dipping their toes into the world of machine learning or are you offering advanced workshop for industry practitioners? Tailoring your content accordingly ensures relevance.
- Content Quality: Invest time in creating high-quality material. A well-structured course with interactive elements tends to attract more students and fosters better learning. Visual aids and real-world applications can enhance engagement.
- Certification Options: Adding certification can also add value to your courses. People love credentials that they can showcase on LinkedIn or CVs. Make your course stand out by offering recognized certifications through collaborations.
Delivering courses, though immensely rewarding, requires not only expertise but also marketing acumen to draw students in. However, once a solid reputation is built, the income generation potential is boundless.
Creating Content for Educational Websites
The digital age has opened avenues for those who have a knack for writing or creating visual content related to machine learning. Websites looking for educational material on this topic are plentiful. From blogs to online magazines, the demand for insightful and well-researched content is insatiable.
- Guest Posts: Many educational websites offer compensations for guest articles. As a contributor, you can share your insights or tutorials, gaining visibility while also earning.
- Tutorials and Case Studies: Detailed case studies and step-by-step tutorials can be beneficial. They not only provide valuable information but also establish you as an authority in the field.
- YouTube and Video Content: Small bite-sized video tutorials can also capture a wider audience. Visual learners often prefer video over text, so putting your knowledge out there in a different format can broaden your reach.
This kind of content creation fosters connections with a community of learners while providing the opportunity to monetize your expert knowledge in machine learning.
Building a Personal Brand Online
Personal branding is about presenting a cohesive and authentic image of yourself in the digital space. In machine learning, a strong online presence can open up doors to collaborations, job offers, and speaking engagements.
- Social Media Interaction: Engaging with communities on platforms like Twitter, LinkedIn, or Facebook can enhance your visibility. Sharing insights, participating in discussions, or even tweeting complex ideas in simpler terms helps in forging connections.
- Portfolio Websites: Build a portfolio that highlights your projects, case studies, and any courses or workshops you've offered. This could serve as an online resume.
- Networking: Engaging in online groups and forums related to machine learning, such as Reddit or specific Facebook groups, can add people to your network who might be looking for freelance work or consultancy.
Creating a personal brand is an ongoing process, but it pays dividends by establishing your place in the machine learning community and widening your financial opportunities over time.
In a world where visibility can translate directly to opportunity, investing time into building your online presence is a step you won't regret.
Networking and Community Engagement
In today's fast-evolving tech landscape, networking is not just a nice-to-have; it's an essential aspect of advancing one's career, particularly in machine learning. By engaging with the community, individuals can leverage collective knowledge, seize new opportunities, and foster collaborations that may otherwise remain isolated. The importance of networking cannot be overstated. It's the bridge that connects talent with opportunity and innovation with application.
Participation in Conferences and Meetups
Conferences and meetups serve as platforms for professionals to socialize and share ideas. Attending these events can be a game changer for anyone involved in machine learning. It offers a chance to learn from industry leaders, participate in workshops, and keep abreast of the latest trends.
Being present at events like the NeurIPS or SIGKDD is invaluable; however, it's not just about showing up. Engaging in discussions and asking questions can lead to deeper insights. Have you ever noticed how some of the most promising collaborations stem from informal conversations at these gatherings? This underscores the notion that being present can indeed lead to unforeseen opportunities.
- Skill Enhancement: Workshops often provide hands-on experiences that can enhance one’s practical skills.
- Knowledge Exchange: Participants showcase their projects, allowing others to learn and critique.
- Networking Opportunities: Meeting like-minded individuals opens doors for potential partnerships.
Furthermore, often such events feature activities like lightning talks. These short presentations mean quick engagement, and they provide fertile ground for discussions.
Contributing to Open Source Projects
Engaging in open-source projects is another effective way to network within the machine learning ecosystem. It’s not just about coding; it’s about collaboration. Whether you're contributing to well-known projects like TensorFlow or Scikit-learn, you’re also adding your name to a list of contributors who share a common vision.
Participating in open source projects offers several benefits:
- Portfolio Enhancement: Showcasing contributions serves as a testament to your skills.
- Learning Opportunity: Working alongside seasoned developers can deepen your understanding.
- Community Building: It fosters relationships that may lead to job offers or collaborations.
When you engage on platforms like GitHub, you're not only gaining visibility but also establishing credibility.
Building Professional Relationships
Once connections have been made, it's vital to nurture those relationships. Professional networking isn’t just collecting contacts; it’s about building meaningful relationships that can lead to mutually beneficial outcomes. To do so effectively, keep these points in mind:
- Follow Up: After meeting someone, send a quick message acknowledging your conversation.
- Be Helpful: Offer your skills when you can; it's a great way to establish goodwill.
- Stay in Touch: Regular check-ins can keep lines of communication open.
Networking is like gardening; you plant the seeds of connection which require care and attention to thrive. As you cultivate these relationships, you not only grow your professional network but also increase your chances of discovering new opportunities in the machine learning field.
"Your network is your net worth."
In summary, engaging with the machine learning community through conferences, open-source contributions, and fostering professional relationships is vital for both personal and career growth. The fruits of networking often manifest in ways one could hardly predict, leading to significant advancements in one's journey within the field.
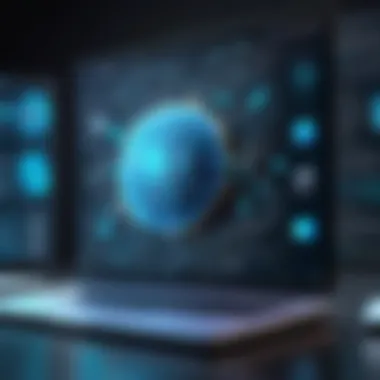
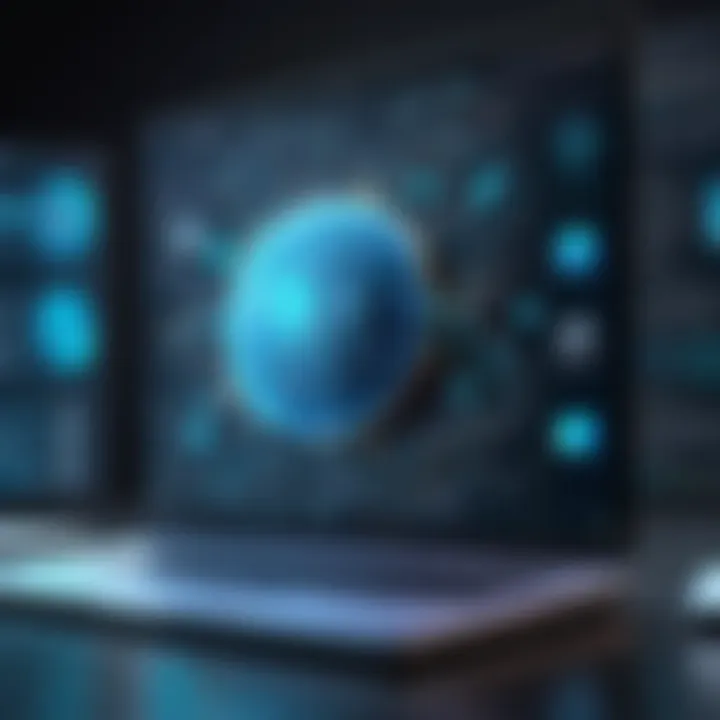
Challenges and Ethical Considerations
When diving into the realm of machine learning, a myriad of opportunities awaits, but it's paramount to also recognize the shadows lurking behind the shiny potential. The ethical considerations and challenges present in this domain are not just regulatory tasks; they are foundational issues that can determine success or failure in monetizing machine learning efforts. This section addresses why understanding these challenges is crucial for those aiming to capitalize on machine learning skills while maintaining integrity and responsibility in their practices.
Addressing Bias in Machine Learning Models
Bias in machine learning models is one of the most discussed problems in today’s tech landscape. Machine learning algorithms learn from data, but if that data reflects societal biases, the algorithms can unconsciously replicate and magnify them. From hiring tools prioritizing certain demographics to credit scoring systems unfairly penalizing individuals based on race, the implications can be severe.
Addressing this bias involves a few key steps:
- Data Auditing: Regularly reviewing datasets for imbalance or prejudice is critical. Before utilizing data, ask questions like, "Who is represented here?" and "Who might be excluded?" A thorough examination can uncover troublesome trends.
- Model Testing: After deploying a model, continuous testing is needed to see how it performs across different groups. If one group receives consistently poorer outcomes, changes should be made.
- Algorithmic Fairness: Incorporating fairness metrics when developing models can guide adjustments. For instance, adjusting weights in an optimization process can help balance outputs for different demographic groups.
"When creating machine learning models, ethical responsibility should be a primary output parameter, not an afterthought."
Creating unbiased algorithms not only promotes fairness but also enhances the broader adoption of technologies by making outputs more reliable and acceptable. Additionally, from a monetary standpoint, companies that ignore bias run the risk of backlash and reputational harm, which can easily impact their bottom line.
Navigating Industry Regulations
Regulatory landscapes are ever-shifting, especially surrounding machine learning. Depending on the industry, challenges range from data protection laws like GDPR to the ethical usage of AI in sectors such as finance and healthcare. Keeping abreast of laws is not just about compliance; it’s about understanding the implications for monetization strategies.
Navigating these regulations involves:
- Staying Informed: Following reputable sources that cover changing regulations can equip you to handle challenges swiftly. Websites like Wikipedia and Britannica offer foundational articles on the topic.
- Building Compliance Checkpoints: Instead of treating regulations as mere roadblocks, see them as checkpoints. Integrating these checkpoints into your workflow can establish a smoother progression from project inception to deployment.
- Consulting Legal Experts: Depending on the complexity and scope of your machine learning projects, involving legal counsel who understands tech law can save time and reduce risk in the long run.
Adhering to industry regulations is not just crucial for avoiding penalties; it is also a competitive advantage. Organizations that proactively comply often find themselves earning consumer trust and loyalty, which are invaluable assets in any monetization strategy.
In summary, addressing bias and navigating regulatory landscapes are not merely chores for machine learning professionals. They represent critical components that can solidify a foundation for ethical monetization practices. In the world of AI, ensuring fairness and compliance could be the key difference between a fleeting opportunity and a sustainable income source.
Resources for Continuous Learning
In an ever-evolving field such as machine learning, staying current is no small feat. Technologies advance like the speed of light and methodologies that were groundbreaking a few years ago can quickly become outdated. For individuals aiming to monetize their skills in this domain, continuous learning is paramount. This not only cultivates expertise but also enhances marketability and ensures that professionals can deliver cutting-edge solutions. Here, we will explore vital resources that can help anyone deepen their knowledge in machine learning, thus paving the way for financial opportunities.
Recommended Literature and Journals
Books and academic journals serve as the bedrock of foundational and advanced knowledge in machine learning. They offer insights into theory, practice, and the latest developments that can sharpen one's skills. Consider diving into the following recommendations:
- “Hands-On Machine Learning with Scikit-Learn, Keras, and TensorFlow” by Aurélien Géron: This hands-on guide covers many practical aspects of machine learning, making it easy to follow for both novices and experienced practitioners.
- “Pattern Recognition and Machine Learning” by Christopher Bishop: This text delves into the statistical principles underlying machine learning—perfect for those who aim to master the math behind algorithms.
- Journals such as the Journal of Machine Learning Research (JMLR): These publications provide peer-reviewed articles that keep readers updated on the latest trends and breakthroughs.
These resources not only build a robust foundation but can also drive innovative thinking—essential for crafting profitable solutions.
Online Courses and Certifications
Navigating online courses can be like trying to pick a needle out of a haystack; however, finding the right ones can significantly enhance your skill set. The flexible nature of these courses allows learners to study at their convenience, making them a fitting choice for busy professionals and students alike. Here are some platforms and certifications worth considering:
- Coursera: Offers programs in partnership with renowned universities. For example, the Machine Learning specialization by Andrew Ng from Stanford University is a popular choice among learners.
- edX: Known for its professional certificate programs. The MicroMasters program in Artificial Intelligence from Columbia University is particularly noteworthy for its depth.
- Udacity: The Nanodegree programs in Machine Learning Engineer or Data Scientist are recognized by industry leaders and can significantly boost one's employability.
The certifications obtained can act like a golden ticket, often demanded by employers, elevating a resume above others in a competitive job market.
Investing in these resources and continually developing your skills can significantly expand your understanding of the field and open doors to lucrative career pathways.
In summary, embracing both literature and online learning as a means to upgrade your knowledge is not simply beneficial; it's essential. By incorporating various learning tools, you not only enhance your competencies but also position yourself as a valuable asset when entering the job market or venturing into freelance work.
Ending: The Future of Machine Learning and Income Generation
As we look ahead, the fusion of machine learning and income potential paints a promising picture. It's not just a passing fad, but a paradigm shift that influences industries, economies, and individual careers. The insights gleaned throughout this article underscore the myriad ways machine learning is becoming integral to business models and personal growth strategies.
Long-term Viability of Machine Learning Careers
When it comes to job stability in the tech world, machine learning careers stand tall. The landscape is rife with opportunities, but it takes a bit of finesse to navigate. Companies are on the hunt for talent who not only understand algorithms but can also apply them to real-world challenges. Research indicates that the demand for machine learning professionals is expected to surge.
"The U.S. Bureau of Labor Statistics anticipates a 31% growth rate for data science and machine learning jobs between 2019 and 2029, much higher than the average for all occupations."
With industries expanding their data capabilities, roles in machine learning are increasingly vital. Whether it’s automating predictions in finance, enhancing customer service through chatbots, or creating intelligent applications, the avenues are vast. This isn’t just a trend; it represents a long-term investment in skills that are bound to be in high demand for years, perhaps decades, to come. The adaptability of machine learning tools also means that professionals must continually update their knowledge, but for those willing to invest the effort, the rewards can be considerable.
Final Thoughts on Deciding to Monetize Skills
The decision to monetize your skills in machine learning is no small feat. It’s necessary to weigh the pros and cons carefully, considering your own unique strengths and interests. While the draw of financial gain is significant, it’s vital to ground that pursuit in a genuine passion for the technology and its applications.
If you're keen to embark on this path, think strategically about how to align your education and experience with market needs. Maybe it's developing a niche product that addresses specific issues, or it could involve offering consulting services to help businesses transition into data-driven decision-making.
Success in this realm often comes from a combination of continuous learning, networking, and practical application of skills.
Here are some considerations:
- Identify Your Niche: What unique skills do you possess? Finding what sets you apart can give you a competitive edge.
- Build Your Portfolio: Start working on projects, even if they're small. Showcasing real-world applications of your skills can attract clients and employers alike.
- Stay Updated: The field evolves quickly. Keeping up with the latest tools and techniques is essential.
In summation, monetizing machine learning skills isn’t just about the numbers. It’s about positioning yourself at the forefront of a technological revolution that’s reshaping how we work, live, and think. With the right mindset and approach, the future can indeed be bright.
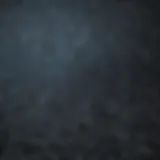
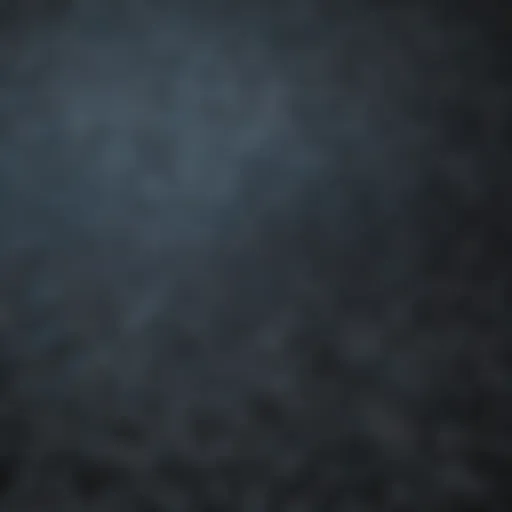