Understanding the Steps in the Machine Learning Process
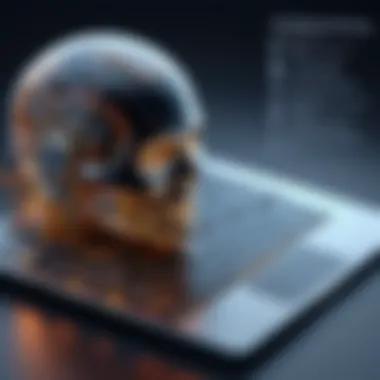
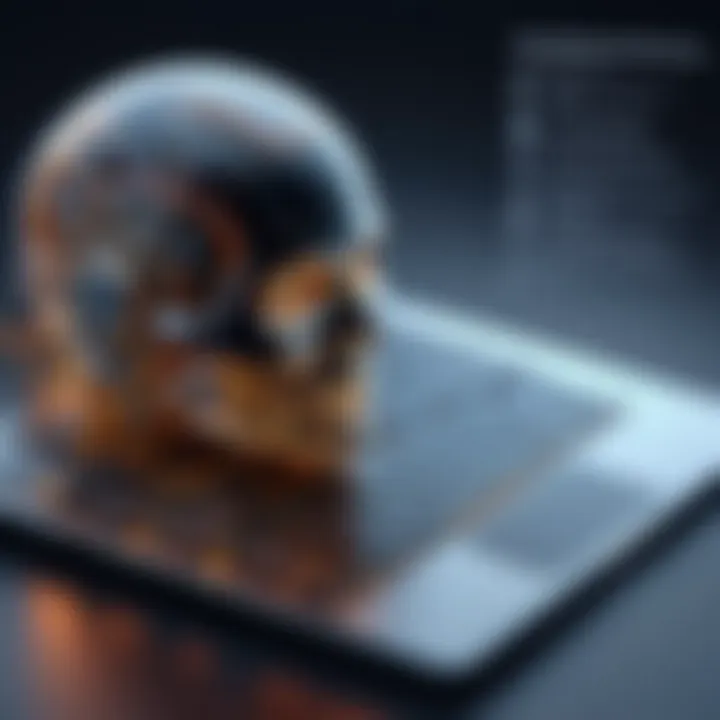
Intro
The journey into machine learning begins much like any significant endeavor: with curiosity and a desire to solve problems. As individuals or organizations venture into this realm, they often encounter a winding path filled with various methodologies and processes. From the initial gathering of data to the meticulous phase of deploying and maintaining a model, understanding each step is crucial. Not only does this knowledge assist in implementing effective solutions, but it also allows practitioners to navigate the complexities involved in the data-driven landscape critically.
As the machine learning domain continues to evolve, gaining insights into the process becomes even more pertinent. Developing systems that learn from data requires more than just technical know-how; it thrives on a well-structured approach that intertwines theory with practical applications. Let's embark on an illuminating exploration of the steps involved in the machine learning process, breaking down the layers that make this field both intricate and rewarding.
Intro to the Machine Learning Process
In the world of technology, machine learning has become a linchpin that holds together various innovations. Understanding the machine learning process is vital for anyone looking to harness its power, whether you're an aspiring programmer, a seasoned IT professional, or a curious technology enthusiast. This segment of the article serves as a guide to help you navigate through each step, culminating in the realization of effective machine learning solutions.
Defining Machine Learning
Machine learning can be defined as a subset of artificial intelligence where systems learn from data, improving over time without being explicitly programmed. Essentially, it’s about creating algorithms that can identify patterns and make decisions based on data inputs. Imagine teaching a child to differentiate between various fruits; they learn from examples, becoming better with each piece of information. Similarly, machines rely on statistical methods to learn from huge datasets.
For instance, think of a scenario where an algorithm is trained to recognize images of cats and dogs. Using thousands of labeled pictures, the model learns to distinguish the two by analyzing features like fur color, shape of ears, and even size. This is the crux of machine learning—using data to fit models that can make predictions or draw insights autonomously.
Importance of the Process
Consider the following benefits of a structured machine learning process:
- Enhanced Decision-Making: A clear understanding of machine learning allows businesses to make data-driven decisions, leading to more accurate outcomes.
- Increased Efficiency: Knowing how to efficiently process data can significantly cut down the time needed to implement effective solutions.
- Scalability: A thorough grasp of the machine learning landscape helps in designing scalable models that can adapt as new data comes in.
"Harnessing machine learning can turn raw data into actionable insights, providing businesses with a competitive edge."
Moreover, a well-defined machine learning process fosters collaboration among teams—data scientists, engineers, and business analysts can work together more effectively if they share an understanding of each step. In such a rapidly evolving field, continuous learning becomes a lifelong commitment; therefore, a step-by-step approach not only demystifies complexities but also leads to greater innovation.
In summary, a solid foundation in machine learning processes serves as the bedrock for advancing both theoretical knowledge and practical application, paving the way for future endeavors in this exciting domain.
Problem Definition
In the realm of machine learning, defining the problem is akin to laying the foundation before constructing a house. Without a solid base, you might find yourself in a precarious situation further down the road. Problem definition acts as the guiding star, steering the direction of the entire project. To embark on a successful journey in machine learning, one can't afford to overlook this crucial step. It's where you identify what you are aiming to achieve and why it matters. This phase not only shapes the project's objectives but also influences how data will be gathered and which algorithms will ultimately be employed.
Identifying the Business Problem
Defining the business problem lays the groundwork for understanding the context in which the machine learning solution will operate. Here, it’s essential to narrow down the specific issue that needs addressing. This might range from analyzing customer churn in a subscription service to predicting stock prices.
Often, the challenge lies in articulating the problem in a way that resonates with stakeholders. A well-defined problem statement should succinctly outline the project goals while tying them directly to business outcomes. For example, instead of stating, "We need to predict something", a more concrete approach would be, "We need to reduce customer churn by at least 15% over the next quarter."
Key considerations for identifying the business problem include:
- Understanding Stakeholder Needs: Engage with decision-makers and end-users to grasp their pain points.
- Feasibility and Impact: Assess whether the problem can realistically be solved with machine learning and the potential impact on the business.
- Scope and Clarity: Clearly define what success looks like—this will serve as a reference point throughout the project.
Establishing Success Metrics
With a business problem sensibly identified, the next step is to define how success will be measured. Establishing success metrics provides a quantifiable means to evaluate the effectiveness of the machine learning model. Success metrics won't just tell you whether the model works; they will also help frame subsequent discussions on model improvement and operational impact.
When selecting success metrics, keep these considerations in mind:
- Alignment with Business Goals: The metrics should directly tie back to the objectives set out when defining the business problem. For example, if the goal is to increase sales through personalized recommendations, metrics like conversion rates or average order value would be appropriate.
- Quantifiability: Choose metrics that can be easily tracked and documented. Clear numbers allow for transparent evaluations.
- Actionability: The metrics should guide future decision-making. They must convey whether adjustments or enhancements are needed.
Some common metrics used in machine learning projects include accuracy, precision, recall, F1 score, and AUC-ROC for classification tasks, while RMSE (Root Mean Square Error) and MAE (Mean Absolute Error) can be used for regression models.
"Defining the problem correctly is half the battle won. It’s easier to build a solution when you know exactly what you’re solving for."
In summary, problem definition is not just a checkbox item in the machine learning process. It is an essential phase that creates a clear blueprint. By carefully identifying the business problem and establishing meaningful success metrics, practitioners position themselves for a smoother and more effective execution of their machine learning projects.
Data Collection
Data collection is the backbone of any machine learning project, serving as the crucial first step before diving into the complexities of algorithms and model training. Without quality data, even the most advanced algorithms are like a car without wheels—unable to move forward. The significance of this phase cannot be understated, especially as it lays the groundwork for all subsequent stages of the machine learning process.
Why is Data Collection Important?
A well-structured data collection process ensures that your model is trained on relevant and high-quality information. This not only improves the accuracy of predictions but also minimizes biases that could skew results. Furthermore, different types of tasks—be it classification, regression, or clustering—demand varying data inputs. Therefore, understanding what data is needed and how to gather it is paramount.
Sources of Data
When talking about sources, it’s crucial to note that data can come from various avenues. Each source brings its unique attributes and challenges. Here are some common data sources:
- Public Datasets: Websites like Kaggle and UCI Machine Learning Repository offer a wealth of datasets for numerous applications. This is a great point for beginners to gather data for their projects.
- APIs: Platforms like Twitter, Facebook, or Google provide APIs that allow you to scrape data efficiently. For instance, using the Twitter API, you can collect tweets related to specific hashtags or events.
- Surveys: If you need data tailored to specific needs, surveys can be an effective way to gather insights. Tools like Google Forms can simplify this process.
- Web Scraping: Creating scripts to pull data from websites can be both effective and efficient when public datasets aren’t available. However, it is essential to respect website terms of service.
- In-House Data: Companies often have valuable internal data that can be critical for training purposes. However, this can involve legal and ethical considerations regarding data privacy.
Data Types and Formats
Understanding the types of data you are dealing with is just as important as knowing where it comes from. Data can typically be categorized into several formats:
- Structured Data: This includes organized information that can be easily entered into a database, like data in tables where each row corresponds to a specific record.
- Unstructured Data: This type comprises raw information that isn’t categorized or organized. It includes text, images, videos, and even audio files. Sorting this can be a challenge but is often where the interesting insights lie.
- Semi-Structured Data: This is a mix between structured and unstructured data, such as XML or JSON files, which contain tags or markers to separate semantic elements.
In addition to data types, the format also plays an essential role. Various formats like CSV, JSON, and XML have different structures and implications for how machine learning models handle them.
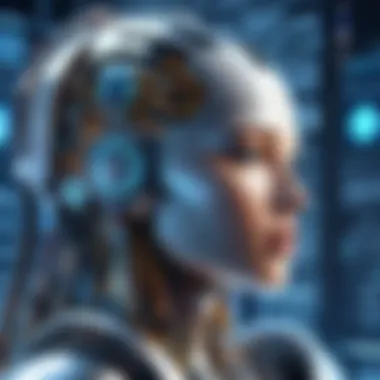
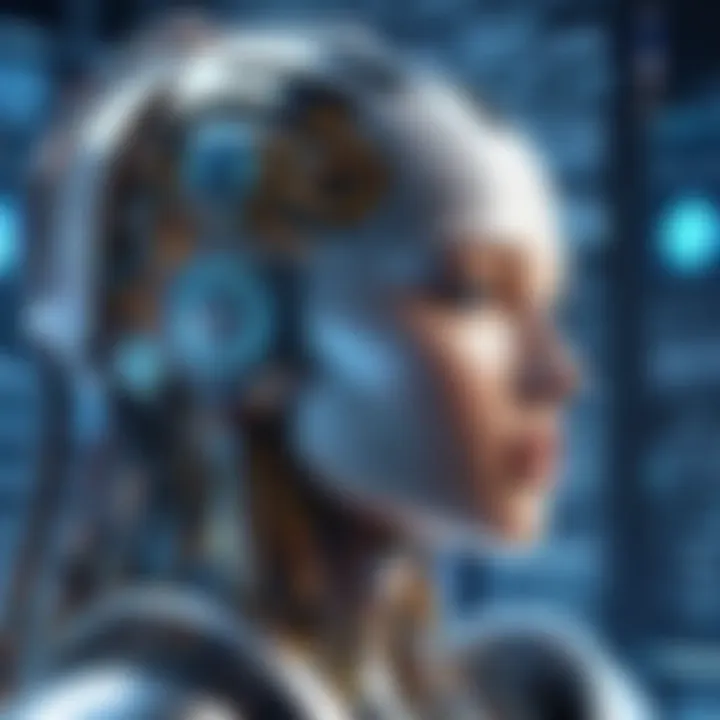
"The best data is useless without the right context, and the wrong context can twist the most well-structured data into a big knot."
In sum, data collection isn’t just about amassing information; it’s about gathering the right information in the right format. By honing in on the sources and types, you empower your machine learning models to operate efficiently—and that’s what ultimately drives success.
Data Preparation
Data preparation is a critical phase in the machine learning process. It's where raw data starts to morph into something manageable and useful. In a world where data is abundant, the importance of preparing it properly can't be overstated. Think of this step as laying a solid foundation before building a house. Without that groundwork, even the most sophisticated model may crumble under its own complexity.
Key Elements in Data Preparation
The journey of data preparation typically involves several specific steps: cleaning, transforming, and engineering data. Each of these steps plays a vital role in ensuring the quality and integrity of the dataset used for modeling.
Cleaning the Data
Cleaning the data is often an arduous yet essential task. Raw data can be rife with irregularities—missing values, duplicates, outliers, and even errors can lurk in the folds. Addressing these issues is paramount. A dataset that's been properly cleaned not only enhances the performance of the model but can also lead to more accurate insights.
The cleaning process can be broken down as follows:
- Identifying Missing Values: Determine how to handle gaps in the data, whether through imputation or exclusion.
- Removing Duplicates: Ensure that each data point is unique to prevent skewed results.
- Outlier Detection: Identify anomalies that could distort model learning.
Effective data cleaning sets the stage for all subsequent steps. Poor data quality can lead to misleading conclusions, which means that practitioners must roll up their sleeves and get their hands dirty with this fundamental step.
Transforming and Normalizing Data
Once cleaning is complete, the next step is transforming and normalizing the data. Transformation alters the data’s format or structure while normalization ensures that different datasets can be compared meaningfully. This is where the magic of preparing features begins.
- Normalization: Adjust numeric data to a common scale, essential for algorithms sensitive to the scale of data like k-nearest neighbors. For instance, rather than numbers that range from 0 to 1, normalization often helps in transforming features into a standard format.
- Encoding Categorical Variables: Transforming non-numeric categories into a format that can be provided to the modeling algorithms. Methods like one-hot encoding or label encoding are often employed.
- A sample method for normalization can be using Min-Max scaling, which rescales the data to fit between specified bounds.
Transformations can reveal patterns that were not visible with raw data, so they are vital. When data is properly normalized and transformed, it leads to more accurate predictions.
Feature Selection and Engineering
Feature selection and engineering represents the pinnacle of the data preparation stage. This process involves selecting the most important features while disregarding irrelevant ones. More importantly, it’s about understanding the problem domain and creatively crafting new features that might unlock deeper insights.
- Selecting Features: It’s crucial to identify which features have significance and potential influence on the outcome. Techniques like recursive feature elimination or the Random Forest feature importance can provide guidance here.
- Engineering New Features: Creating new variables from existing ones can enhance model performance. For example, if you have a 'date' feature, deriving features like 'day of the week' or 'month' could provide valuable insights for prediction.
Overall, thoughtful feature engineering captures the nuances of the data, allowing models to learn more effectively. As any experienced machine learning practitioner knows, sometimes a well-engineered feature is the secret sauce that elevates a model from mediocre to exceptional.
Proper data preparation is the bedrock of high-performing machine learning models. Neglecting this step can lead to failure at later stages, no matter how advanced your algorithms are.
Model Selection
Model selection stands as a critical phase in the machine learning process, functioning as a bridge between the theoretical understanding of algorithms and their practical application. This step not only influences the model's ultimate performance but also defines how well it can solve the specific problem at hand. Choosing the right model involves a careful evaluation of various factors: the nature of the data, the complexity of the task, and the desired outcomes. It's akin to selecting the right tool for a job—every tool has its purpose, and the same goes for algorithms in machine learning.
Consider this: an algorithm successful in one scenario might falter in another due to varying data characteristics or inherent challenges of the problem. Practitioners must thoughtfully navigate through this selection process. Often, this involves multiple iterations and adjustments before settling on a model that not only addresses the immediate needs but also aligns with long-term goals. With an eye for detail, one can see how this step can create an avenue for innovation and adaptability in building intelligent systems.
Understanding Different Algorithms
Algorithms in machine learning can be broadly categorized into three major types: supervised, unsupervised, and reinforcement learning. Each has its unique advantages and applicability, and understanding these distinctions is paramount.
- Supervised Learning: This approach involves learning a function that maps inputs to outputs based on labeled data. Common algorithms include Logistic Regression, Decision Trees, and Neural Networks. Supervised learning shines in scenarios where historical data is available, offering reliable predictions for unseen data.
- Unsupervised Learning: In contrast, this type of learning deals with unlabeled data. Algorithms like K-means Clustering and Hierarchical Clustering help identify structures or patterns without any prior knowledge of the output. It's useful for exploratory analysis and is often employed in customer segmentation and anomaly detection.
- Reinforcement Learning: This method, often seen in game-playing AI, involves agents learning to make decisions by taking actions in an environment to maximize cumulative rewards. It’s particularly suited for problems requiring a sequence of actions.
The understanding of these categories is just the tip of the iceberg. Within each category lies a menagerie of algorithms, each with unique tuning parameters and operational nuances. Researchers and developers must delve deeper, studying the behavior, strengths, and weaknesses of algorithms to determine which fits best with their specific dataset and objectives.
Choosing the Right Model
Selecting the right model isn't merely a technical choice; it demands an understanding of the data and the problem’s context. Here are several key considerations:
- Data Characteristics: The features of the dataset can dictate which algorithms are promising. For instance, high-dimensional data might favor algorithms like Support Vector Machines that can excel in such environments.
- Problem Complexity: Simple relationships can often be modeled effectively with linear approaches, while complex, non-linear relationships may require advanced methods like ensemble techniques or deep learning.
- Computational Resources: Some models require more computational power than others. A decision tree may run quickly, while deep learning models tend to need significant processing time and memory.
- Interpretability vs. Performance: There’s often a trade-off between the interpretability of the model and its predictive performance. A highly accurate model that functions as a black box might not satisfy stakeholders requiring clear reasoning behind decisions. Hence, transparency and ease of interpretation can play a crucial role in the model selection phase.
In summary, the model selection process weaves together the threads of problem understanding, data exploration, and algorithm capabilities. Practitioners are encouraged to engage in systematic comparisons, potentially utilizing tools like cross-validation to gauge model performance. By being judicious and informed during this selection process, one lays a robust foundation for the forthcoming stages, propelling the project towards success within the dynamic landscape of machine learning.
Model Training
Model training is a critical phase in the machine learning process. It serves as the pivotal point where insights derived from data begin to morph into usable models. Without proper training, even the best-designed algorithms can end up floundering. The importance of this step cannot be overstated. This is where patterns are discovered, and the model learns to make predictions based on the data provided.
Training the Model
Training the model involves feeding it a dataset, allowing the algorithm to learn from this data. Typically, training sets consist of input features and output labels. For example, if we were building a model to predict house prices, the input features might include square footage, the number of bedrooms, and neighborhood quality. The output would be the respective prices of those houses.
It’s crucial that this dataset is both comprehensive and representative. Relying on a hodgepodge of limited data will result in a model that doesn’t generalize well to new scenarios. The quality of training data heavily influences the model’s performance, and it's often said that “garbage in, garbage out” holds true in machine learning.
During this process, the algorithm iteratively adjusts its internal parameters based on the errors in its predictions. The objective is to minimize these discrepancies through optimization techniques. Most common methods include gradient descent, which adjusts parameters incrementally based on the derivative of the model's error function. Keep in mind that the training process can sometimes be time-consuming, particularly with large datasets or complex models.
Hyperparameter Tuning
Once the basic model training is set, the focus turns to hyperparameter tuning. Hyperparameters are settings that dictate the structure and behavior of the learning algorithm itself. They differ from regular parameters, which are learned directly from the training data.
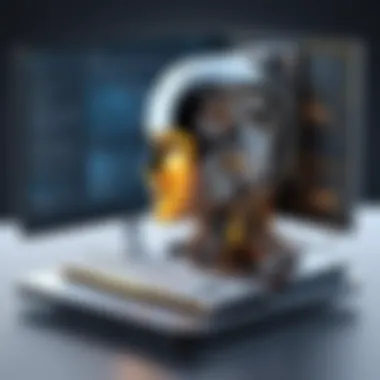
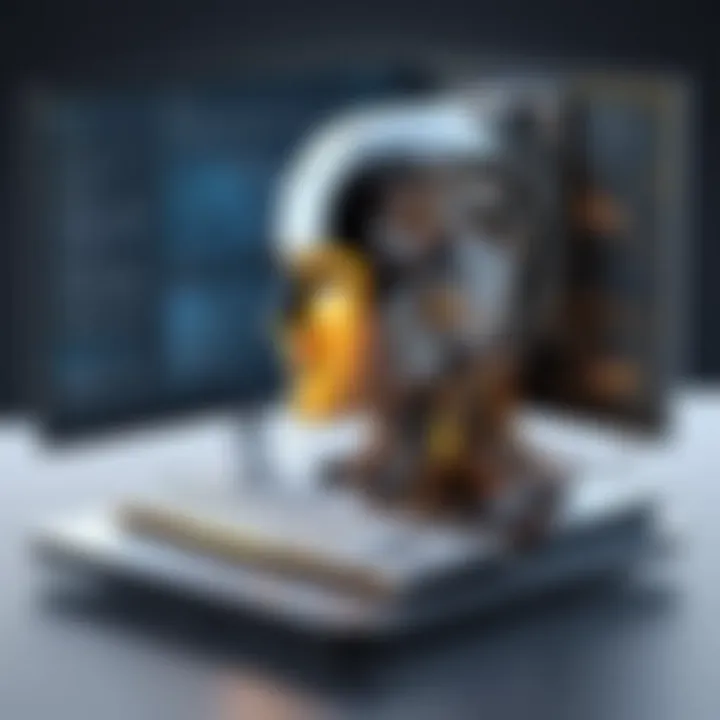
Think of hyperparameters as the knobs and dials that need adjusting to find the sweet spot for optimal performance. For instance, in a neural network, the learning rate and the number of layers can dramatically influence how well the model learns. Choosing the wrong values can result in overfitting or underfitting the data – a cat and mouse game that many practitioners have faced.
Here are a few common hyperparameters one might consider:
- Learning rate: Controls how quickly a model learns. A rate too high can overshoot the optimal parameters, while too low can lead to lengthy training periods.
- Number of iterations: Refers to how many times the learning algorithm will run through the training data.
- Batch size: This dictates how much data the model processes in one go. Adjusting this can balance speed and model robustness.
Hyperparameter tuning requires a systematic approach and could involve techniques such as grid search or random search, each with its own sets of advantages and drawbacks. The goal here is to fine-tune these hyperparameters, enhancing the model’s accuracy and effectiveness.
Ultimately, successful hyperparameter tuning can be a gamechanger, enabling models to achieve performance that may have seemed unattainable with default settings.
In closing, model training is not just a procedural step; it is the crucible where a handful of pixels, numbers, and observations turn into meaningful predictions. The journey from feeding the data into a model to fine-tuning hyperparameters is a blend of art and science, balancing between technical expertise and an uncanny instinct for understanding data.
Model Evaluation
Evaluating a machine learning model is like checking your ingredients before baking a cake. If the ingredients aren't right, the end result could disappoint you. Model evaluation is crucial because it allows developers and researchers to validate their assumptions, test their hypotheses, and ensure that the models perform as expected in real-world scenarios. It’s the stage where you assess whether the model is fit-for-purpose or merely window dressing.
One of the key elements in this process is the distinction between the capabilities of the model and its effectiveness. Having a theoretically sound model isn’t enough. Evaluation sheds light on how well a model generalizes to unseen data, which is ultimately what we want — a well-rounded model that doesn't just memorize patterns from training data but learns to predict outcomes accurately across different datasets.
Performance Metrics
When it comes to performance metrics, it’s like grading a student. The grades you choose to assign should appropriately reflect their understanding of the subject. In machine learning, the metrics can depend significantly on the type of problem being solved. For instance, different metrics apply to classification and regression tasks, hence the need to choose wisely.
Some common performance metrics include:
- Accuracy: Discusses how often the model is right, but be wary; accuracy can be misleading in imbalanced datasets.
- Precision and Recall: Crucial for classification problems, these metrics tell you how well your model identifies the positive class and how many actual positives it captures.
- F1 Score: A combination of precision and recall, it helps provide a balance between the two and is especially useful when you want to highlight the importance of false positives and false negatives.
- Mean Absolute Error (MAE) and Mean Squared Error (MSE): For regression models, these give insight into the average errors the model makes, helping to fine-tune performance.
Evaluating models through these metrics allows you to make informed decisions on whether the model is ready for deployment or if further adjustments are needed.
Cross-Validation Techniques
Cross-validation can be likened to a safety net that catches a performer who is attempting a complicated act. It ensures that the model isn’t overfitting – where it learns too much from the training data. With cross-validation, the data gets divided into subsets, allowing the model to be trained on some folds while testing it on others.
Here are some popular techniques:
- K-Fold Cross-Validation: Dividing the data into 'k' groups and training the model 'k' times, each time holding back a different group for testing. This method provides a good understanding of model stability and reliability.
- Stratified K-Fold: Similar to K-Fold but ensures that each fold maintains the percentage of samples for each class, which is particularly useful for imbalanced datasets.
- Leave-One-Out Cross-Validation (LOOCV): It’s like having a very rigorous academic review process. Here, each sample serves as a test case while the rest are used for training. This can be computationally expensive but is thorough.
By employing these techniques, you can have a clearer view of your model's robustness, helping identify if it’s ready to take on real-world tasks or needs more refinement.
Estimating a model's efficacy through these evaluation methods isn’t just beneficial; it’s essential for ensuring long-term success in deployment.
Model Deployment
Model deployment is a vital step in the machine learning process, serving as the bridge between theoretical models and real-world applications. It is the phase where solutions crafted through rigorous data analysis and model training become usable products or services. Without deployment, all prior efforts in data collection, preparation, and modeling would be moot. In essence, deployment is where the rubber meets the road.
By deploying models, organizations can harness the power of machine learning to automate decisions, enhance user experiences, or provide innovations that were previously unimaginable. The importance of this step cannot be understated; it transforms an academic exercise into a practical tool that yields tangible benefits. The landscape of technology is evolving fast, and organizations that successfully deploy their models gain a competitive edge.
Deploying in a Production Environment
When deploying a model into a production environment, careful planning is essential. This stage involves making sure that the model runs smoothly under real-world conditions, often quite different from the development environment where it was built. Here, the focus is on scalability, reliability, and performance.
A few key points to consider:
- Scalability: Can the model handle the volume expected from users? This needs hefty infrastructure consideration. Using cloud services like AWS or Azure often comes in handy, as they can provide the necessary resources when traffic spikes.
- Latency: Users expect immediate results. The time it takes for a model to serve predictions matters a great deal in applications like online shopping or real-time fraud detection.
- Monitoring and Alerts: Once the model is deployed, it’s crucial to have systems to monitor its performance. Set up alerts for any performance dips that could signal data drift or other issues.
By addressing these concerns, organizations ensure a smoother integration of machine learning solutions into their regular operations.
Integration with Existing Systems
Integrating machine learning models with existing systems is another essential consideration during deployment. If new models do not seamlessly align with current workflows, even the best-designed solutions can falter.
Several factors highlight the importance of this integration:
- Compatibility: It’s essential that the machine learning model can work with existing databases, APIs, and user interfaces. For instance, if your model predicts customer purchasing behaviors, it must align with CRM software to provide relevant insights to sales teams.
- Simplifying Workflows: The goal should be to enhance existing systems, not complicate them. Streamlining processes will increase user adoption. This can be done by ensuring that interfaces are intuitive and that the model outputs are actionable for end-users.
- Feedback Loops: Building a feedback mechanism is crucial. Allowing systems to learn from initial model outputs and user interactions can lead to continuous improvement and optimization. This ensures that models remain relevant as the context and data evolve.
Integrating machine learning models with existing systems maximizes their utility, enhancing the overall workflow and driving better results for businesses and users alike.
In deployment, the model ceases to be a project; it becomes a tool, a catalyst for change and innovation within an organization.
Overall, the deployment of models marks a transformative phase in machine learning. It requires meticulous attention to detail, as one misstep could lead to inefficiencies and lost opportunities. Successful deployment sets the stage for continuous improvement and innovation, ensuring that machine learning initiatives yield the insights and automated decisions organizations crave.
Model Maintenance
Model maintenance is a crucial phase in the machine learning process that ensures a model continues to deliver effective results over time. This stage is often overlooked by practitioners who might focus more on the upfront data collection or model deployment phases, but maintaining a model is just as vital. Without regular oversight and updates, a machine learning model risks becoming obsolete or, worse, making inaccurate predictions. This can especially be the case in a world where data is constantly evolving.
Monitoring Model Performance

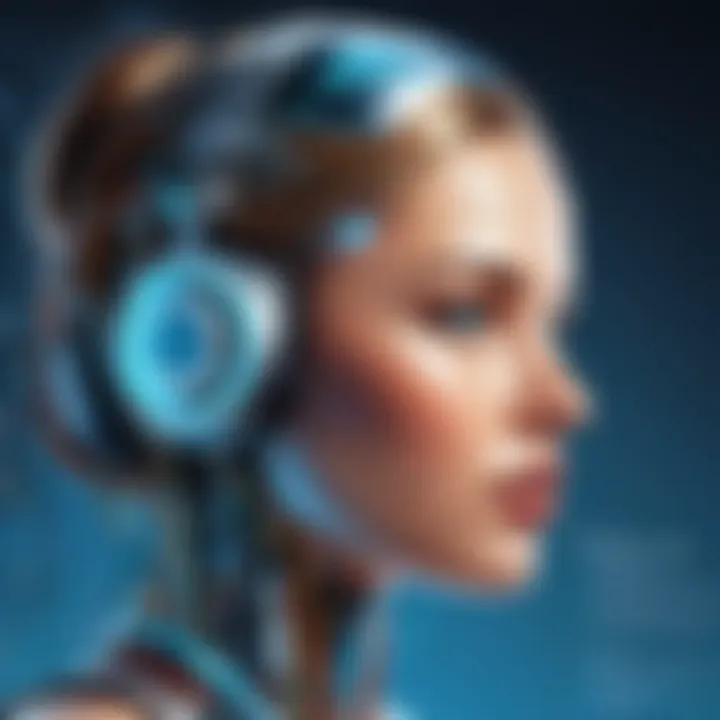
Monitoring model performance refers to the ongoing evaluation of how a model behaves with new data. It's like keeping an eye on the health of an engine; you don't just check it once and forget about it. Regular checks can uncover "drifts" in the data. For instance, if you initially trained a model on data relevant to 2020, and you try to use it again in 2023 without checks, many variables may have changed—market trends, consumer behavior, or even seasonal influences. This is where monitoring comes in.
Here are some essential elements to consider in the monitoring process:
- Performance Metrics: You should consistently evaluate important metrics like accuracy, precision, and recall.
- Data Drift: Watch for changes in the distribution of input data. A sudden shift can indicate that the model isn't performing as expected.
- Feedback Loops: Implementing a feedback system from users can help identify errors and successes in real time.
Regular monitoring allows organizations to track performance trends, catch discrepancies early, and adjust as necessary. Without it, you might just find yourself sitting in a sinkhole, not knowing until it's too late.
Updating the Model
Updating the model is the next logical step following monitoring. As new data comes in or environments change, it becomes essential to refresh the model to reflect these changes. Not updating can seriously hinder performance and lead to misguided decisions.
When considering updating your model, you should keep in mind:
- Retrain with New Data: Incorporating the most recent data can improve the model's accuracy, allowing it to capture the latest trends and insights.
- Adapting to Changes: Sometimes, you'll need to adjust the model architecture or select new features based on shifting patterns in the data.
- Regular Schedule: A timetabled refresh (say quarterly or bi-annually) can serve as a proactive measure, rather than waiting for a huge drop-off in performance.
In the fast-paced realm of technology, no model should be left unattended. Keep the engines running smoothly to avoid unpleasant surprises along the road.
Ethical Considerations in Machine Learning
As machine learning continues to weave its way into various aspects of our daily lives, the importance of ethical considerations grows increasingly apparent. This part of the article isn't just an addendum but a vital component woven throughout the entire process of machine learning. Understanding the ethical implications not only enhances the quality of the models created but also builds trust with users and stakeholders. With advances in technology, a responsible approach to these considerations has become even more paramount.
Bias and Fairness
Bias in machine learning models can be insidious, creeping in through the data used to train algorithms or through the models themselves. The presence of bias can lead to unequal treatment of different groups of people. Consider a scenario where a facial recognition system is primarily trained on images of lighter-skinned individuals. The resulting model may struggle to accurately identify people with darker skin tones, leading to unfair outcomes.
To confront this challenge, it’s crucial to:
- Identify potential biases early, by scrutinizing the data sourcing and preparation stages.
- Utilize diverse datasets to ensure the training process encompasses various demographic groups, ultimately enhancing the generalizability of the model.
- Regularly assess models for biases even after deployment, as user interactions may introduce new forms of data representation that could cause unforeseen disparities.
These steps help forge a path toward fairer machine learning models, but fairness isn't merely a box to check; it’s a continuous commitment to refining processes and improving outcomes for everyone involved.
Transparency and Accountability
In the realm of machine learning, transparency refers to the clarity about how models function and the rationale behind their decisions. This aspect directly ties to accountability – developers and organizations must take responsibility for the outcomes of their algorithms.
Imagine a financial institution using a machine learning model to assess loan eligibility. If an applicant is denied a loan, they should be able to understand the reasoning behind that decision. This is where transparency plays its crucial role.
Here are significant practices that can enhance transparency and accountability in machine learning:
- Documenting the development process: Keeping records of how datasets are selected, how modeling choices are made, and how performance metrics are evaluated ensures traceability.
- Explaining model predictions: Incorporating tools like LIME (Local Interpretable Model-agnostic Explanations) or SHAP (SHapley Additive exPlanations) can aid in elucidating model predictions in a user-friendly manner.
- Engaging with stakeholders: Involving users in discussions about the model's objectives and potential impacts fosters a sense of accountability and can cultivate a collaborative environment for addressing ethical dilemmas.
Future Trends in Machine Learning
As we look ahead, the landscape of machine learning is evolving rapidly, driven by continuous advancements in technology and innovation. This section is crucial to understanding how emerging trends shape the future of machine learning practices. By exploring the latest technologies and the rising integration of AI augmentation, organizations can prepare themselves to harness these developments effectively, leading to more sophisticated tools and solutions.
Emerging Technologies
Emerging technologies are at the forefront of advancing machine learning capabilities. Some noteworthy trends include:
- Quantum Computing: This revolutionary computing method leverages quantum bits (qubits) to process data at unprecedented speeds. Quantum algorithms can solve complex optimization problems significantly faster than traditional computers, which could redefine tasks from training models to data processing, allowing for a leap in predictive capabilities.
- Federated Learning: This decentralized approach allows multiple organizations to collaboration while keeping their data secure and private. Models are trained across numerous devices without sharing sensitive information, making it an invaluable tool for industries where privacy is paramount, like healthcare and finance.
- Edge Computing: By processing data closer to its source rather than relying entirely on central servers, edge computing reduces latency and bandwidth use. This trend is particularly important in Internet of Things (IoT) applications, where real-time data analysis can enhance decision-making on the fly, improving user experience and operational efficiency.
Moreover, advancements in natural language processing and computer vision continue to create smarter applications, affecting various sectors including customer service, autonomous vehicles, and security.
"The rate of technological adoption often parallels the journey of innovation in machine learning, creating endless opportunities for those prepared to adapt."
The Role of AI Augmentation
AI augmentation is becoming increasingly integral to machine learning, enhancing human capabilities rather than replacing them. This synergy between human intelligence and smart algorithms brings several benefits:
- Improved Decision-Making: By leveraging AI tools that analyze vast amounts of data, professionals can make more informed choices faster. For instance, in healthcare, machine learning can identify patterns in patient data, allowing doctors to diagnose conditions earlier.
- Creative Collaboration: AI is not just about numbers and algorithms; it's stepping into creative roles too. By using generative models, artists and creators are being empowered to explore new dimensions of creativity, blending human intuition with computational prowess.
- Scalability and Efficiency: Augmentation helps businesses scale their operations efficiently. Instead of human workers sifting through terabytes of data, AI can handle these tasks, freeing up time for individuals to focus on higher-level strategy and innovation. This results in a more agile and responsive organization.
The interplay between AI augmentation and traditional machine learning stands to enhance both productivity and creativity across varied niches, making the future all the more exciting. The crucial takeaway here is that while technology evolves, the human element remains irreplaceable, emphasizing the importance of a harmonious relationship between man and machine.
End
As we wind down this journey through the machine learning process, it's crucial to recognize the significance of a strong conclusion not only in tying all the loose ends but also in illuminating the broader implications of what has been discussed.
Recap of the Steps
To recap where we've been, the machine learning process is a multi-step affair that starts with a clear problem definition. From there, we dive into the depths of data collection, ensuring we gather the right stuff from diverse sources. Data preparation comes next, where the raw materials are cleaned and transformed into something usable. This leads us to the selection of an appropriate model, which is then meticulously trained. After training, we evaluate the model using various performance metrics to ensure it's fit for deployment.
Each of these steps is interconnected, creating a chain that, when forged properly, results in a robust machine learning solution. Being mindful of the sequence of these actions, and their interdependencies, is essential. Disregarding even one step can lead to unwieldy models that might crash and burn when put into play.
"The success of a machine learning project lies not just in the technology, but in the understanding of the problem and the context in which it operates."
The Ever-Evolving Nature of Machine Learning
Machine learning is not a stagnant field; it is as dynamic as the data it processes. Advances in algorithms, computational power, and data accessibility continually push the boundaries of what is possible.
• Emerging Technologies: Consider the impact of quantum computing as a game-changer for complex computations. • Algorithmic Innovations: New techniques, like transformer models used in natural language processing, illustrate how quickly methodologies can evolve.
This evolution brings both opportunities and challenges. For instance, the increasing availability of data presents a double-edged sword; more data can mean better models, but it can also introduce issues of bias and quality that must be carefully managed.